Towards Mining Latent Client Identifiers from Network Traffic
Authors: Sakshi Jain ([UC Berkeley, LinkedIn]), Mobin Javed (UC Berkeley), Vern Paxson ([UC Berkeley, ICSI])
Volume: 2016
Issue: 2
Pages: 100–114
DOI: https://doi.org/10.1515/popets-2016-0007
Abstract: Websites extensively track users via identifiers that uniquely map to client machines or user accounts. Although such tracking has desirable properties like enabling personalization and website analytics, it also raises serious concerns about online user privacy, and can potentially enable illicit surveillance by adversaries who broadly monitor network traffic. In this work we seek to understand the possibilities of latent identifiers appearing in user traffic in forms beyond those already well-known and studied, such as browser and Flash cookies. We develop a methodology for processing large network traces to semi-automatically discover identifiers sent by clients that distinguish users/devices/browsers, such as usernames, cookies, custom user agents, and IMEI numbers. We address the challenges of scaling such discovery up to enterprise-sized data by devising multistage filtering and streaming algorithms. The resulting methodology reflects trade-offs between reducing the ultimate analysis burden and the risk of missing potential identifier strings. We analyze 15 days of data from a site with several hundred users and capture dozens of latent identifiers, primarily in HTTP request components, but also in non-HTTP protocols.
Keywords: privacy, client identifiers, mining network traffic, tracking
Copyright in PoPETs articles are held by their authors. This article is published under a Creative Commons Attribution-NonCommercial-NoDerivs 3.0 license.
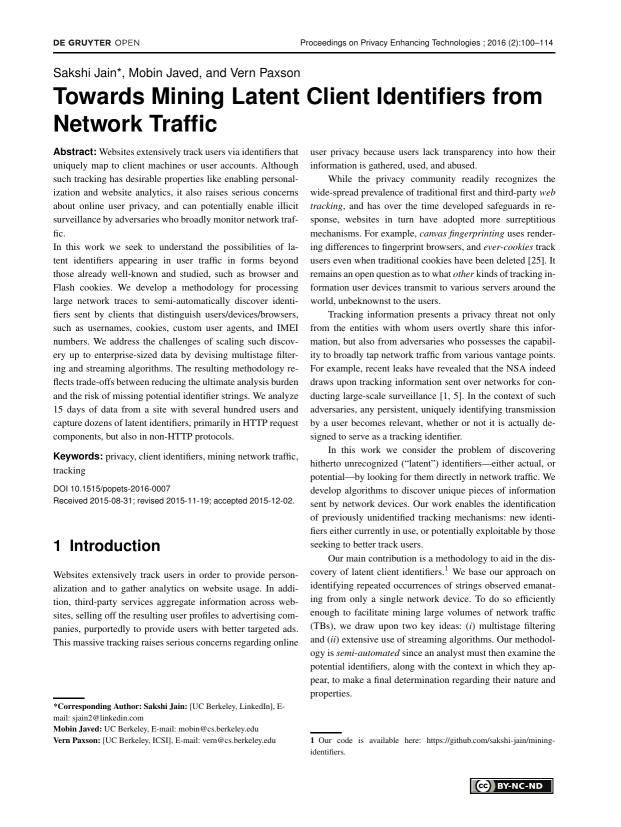