Detecting Anti Ad-blockers in the Wild
Authors: Muhammad Haris Mughees (University of Illinois UrbanaChampaign), Zhiyun Qian (University of California-Riverside), Zubair Shafiq (The University of Iowa)
Volume: 2017
Issue: 3
Pages: 130–146
DOI: https://doi.org/10.1515/popets-2017-0032
Abstract: The rise of ad-blockers is viewed as an economic threat by online publishers who primarily rely on online advertising to monetize their services. To address this threat, publishers have started to retaliate by employing anti ad-blockers, which scout for ad-block users and react to them by pushing users to whitelist the website or disable ad-blockers altogether. The clash between ad-blockers and anti ad-blockers has resulted in a new arms race on the Web. In this paper, we present an automated machine learning based approach to identify anti ad-blockers that detect and react to ad-block users. The approach is promising with precision of 94.8% and recall of 93.1%. Our automated approach allows us to conduct a large-scale measurement study of anti ad-blockers on Alexa top-100K websites. We identify 686 websites that make visible changes to their page content in response to ad-block detection. We characterize the spectrum of different strategies used by anti ad-blockers. We find that a majority of publishers use fairly simple first-party anti ad-block scripts. However, we also note the use of thirdparty anti ad-block services that use more sophisticated tactics to detect and respond to ad-blockers.
Keywords: ad-blockers, anti ad-blockers
Copyright in PoPETs articles are held by their authors. This article is published under a Creative Commons Attribution-NonCommercial-NoDerivs 3.0 license.
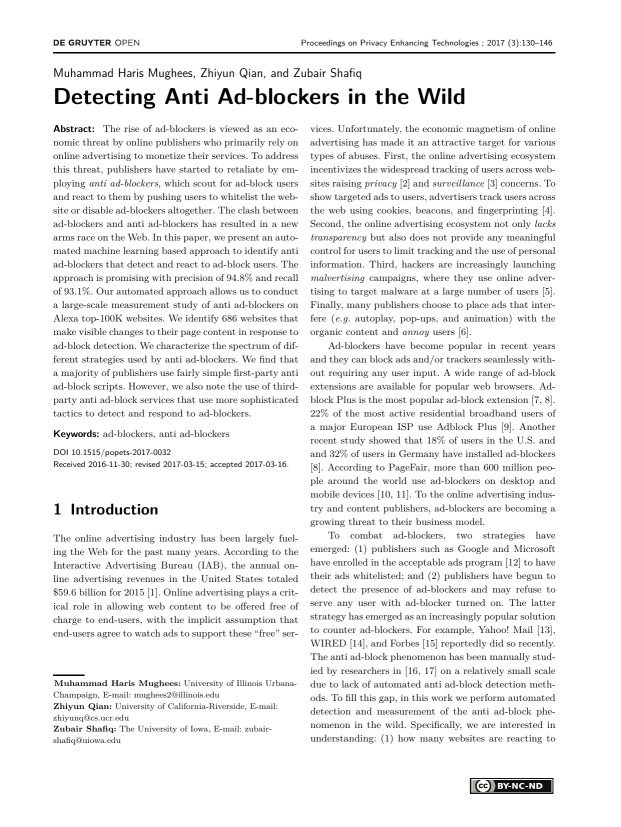