Location Privacy for Rank-based Geo-Query Systems
Authors: Wisam Eltarjaman (University of Denver), Rinku Dewri (University of Denver), Ramakrishna Thurimella (University of Denver)
Volume: 2017
Issue: 4
Pages: 77–96
DOI: https://doi.org/10.1515/popets-2017-0039
Abstract: The mobile eco-system is driven by an increasing number of location-aware applications. Consequently, a number of location privacy models have been proposed to prevent the unwanted inference of sensitive information from location traces. A primary focus in these models is to ensure that a privacy mechanism can indeed retrieve results that are geographically the closest. However, geo-query results are, in most cases, ranked using a combination of distance and importance data, thereby producing a result landscape that is periodically flat and not always dictated by distance. A privacy model that does not exploit this structure of geo-query results may enforce weaker levels of location privacy. Towards this end, we explore a formal location privacy principle designed to capture arbitrary similarity between locations, be it distance, or the number of objects common in their result sets. We propose a composite privacy mechanism that performs probabilistic cloaking and exponentially weighted sampling to provide coarse grain location hiding within a tunable area, and finer privacy guarantees under the principle inside this area. We present extensive empirical evidence to supplement claims on the effectiveness of the approach, along with comparative results to assert the stronger privacy guarantees.
Keywords: location privacy; indistinguishability; rankbased query results
Copyright in PoPETs articles are held by their authors. This article is published under a Creative Commons Attribution-NonCommercial-NoDerivs 3.0 license.
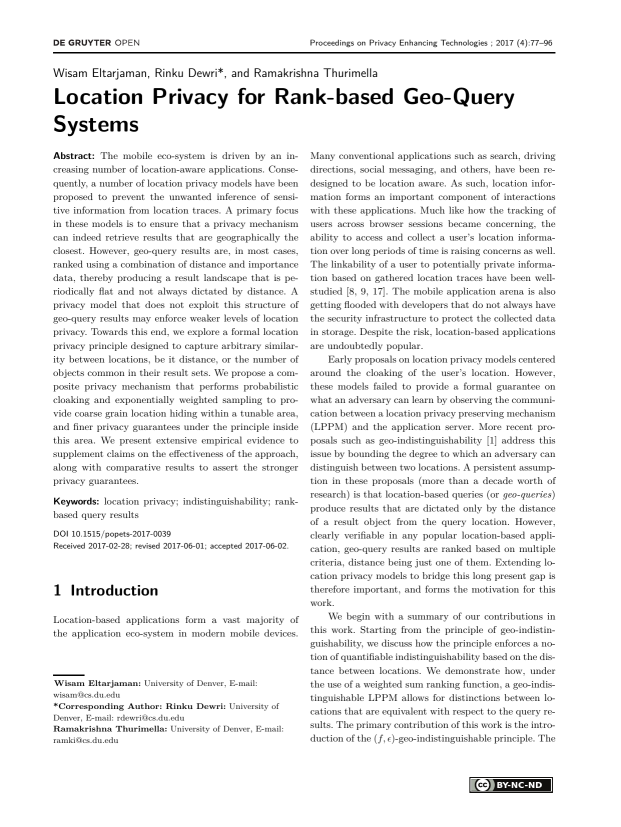