What Does The Crowd Say About You? Evaluating Aggregation-based Location Privacy
Authors: Apostolos Pyrgelis (University College London), Carmela Troncoso (IMDEA Software Institute), Emiliano De Cristofaro (University College London)
Volume: 2017
Issue: 4
Pages: 156–176
DOI: https://doi.org/10.1515/popets-2017-0043
Abstract: Information about people’s movements and the locations they visit enables an increasing number of mobility analytics applications, e.g., in the context of urban and transportation planning, In this setting, rather than collecting or sharing raw data, entities often use aggregation as a privacy protection mechanism, aiming to hide individual users’ location traces. Furthermore, to bound information leakage from the aggregates, they can perturb the input of the aggregation or its output to ensure that these are differentially private. In this paper, we set to evaluate the impact of releasing aggregate location time-series on the privacy of individuals contributing to the aggregation. We introduce a framework allowing us to reason about privacy against an adversary attempting to predict users’ locations or recover their mobility patterns. We formalize these attacks as inference problems, and discuss a few strategies to model the adversary’s prior knowledge based on the information she may have access to. We then use the framework to quantify the privacy loss stemming from aggregate location data, with and without the protection of differential privacy, using two real-world mobility datasets. We find that aggregates do leak information about individuals’ punctual locations and mobility profiles. The density of the observations, as well as timing, play important roles, e.g., regular patterns during peak hours are better protected than sporadic movements. Finally, our evaluation shows that both output and input perturbation offer little additional protection, unless they introduce large amounts of noise ultimately destroying the utility of the data.
Keywords: location privacy; privacy quantification; aggregate locations; inference attacks; differential privacy
Copyright in PoPETs articles are held by their authors. This article is published under a Creative Commons Attribution-NonCommercial-NoDerivs 3.0 license.
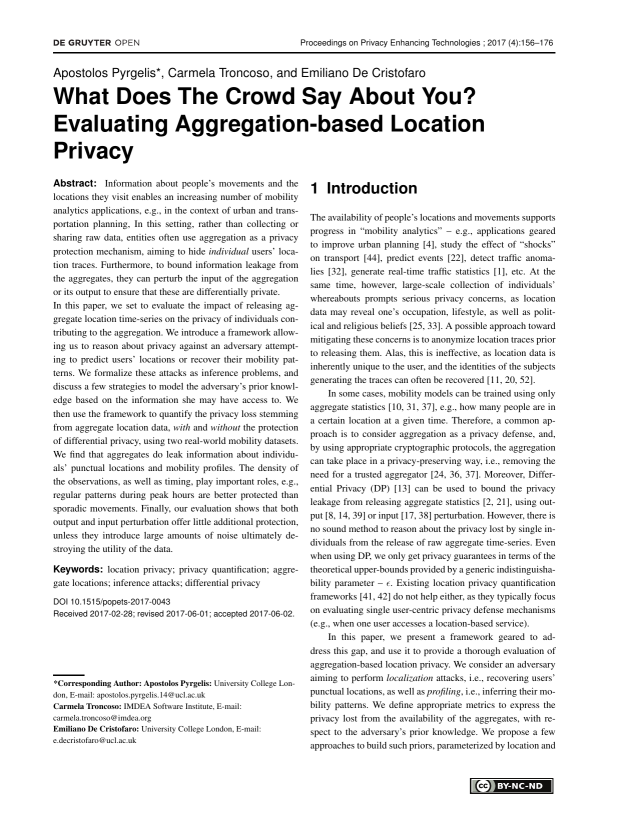