Privacy-preserving Wi-Fi Analytics
Authors: Mohammad Alaggan (Univ Lyon, Inria, INSA Lyon, CITI, Villeurbanne, France), Mathieu Cunche (Univ Lyon, INSA Lyon, Inria, CITI, Villeurbanne, France), Sébastien Gambs (Université du Québec à Montréal (UQAM), Canada)
Volume: 2018
Issue: 2
Pages: 4–26
DOI: https://doi.org/10.1515/popets-2018-0010
Abstract: As communications-enabled devices are becoming more ubiquitous, it becomes easier to track the movements of individuals through the radio signals broadcasted by their devices. Thus, while there is a strong interest for physical analytics platforms to leverage this information for many purposes, this tracking also threatens the privacy of individuals. To solve this issue, we propose a privacy-preserving solution for collecting aggregate mobility patterns while satisfying the strong guarantee of ε-differential privacy. More precisely, we introduce a sanitization mechanism for efficient, privacy-preserving and non-interactive approximate distinct counting for physical analytics based on perturbed Bloom filters called Pan-Private BLIP. We also extend and generalize previous approaches for estimating distinct count of events and joint events (i.e., intersection and more generally t-out-of-n cardinalities). Finally, we evaluate expirementally our approach and compare it to previous ones on real datasets.
Keywords: Physical Analytics, Differential Privacy, Pan Privacy, Randomized Response, Cardinality Set Intersection.
Copyright in PoPETs articles are held by their authors. This article is published under a Creative Commons Attribution-NonCommercial-NoDerivs 3.0 license.
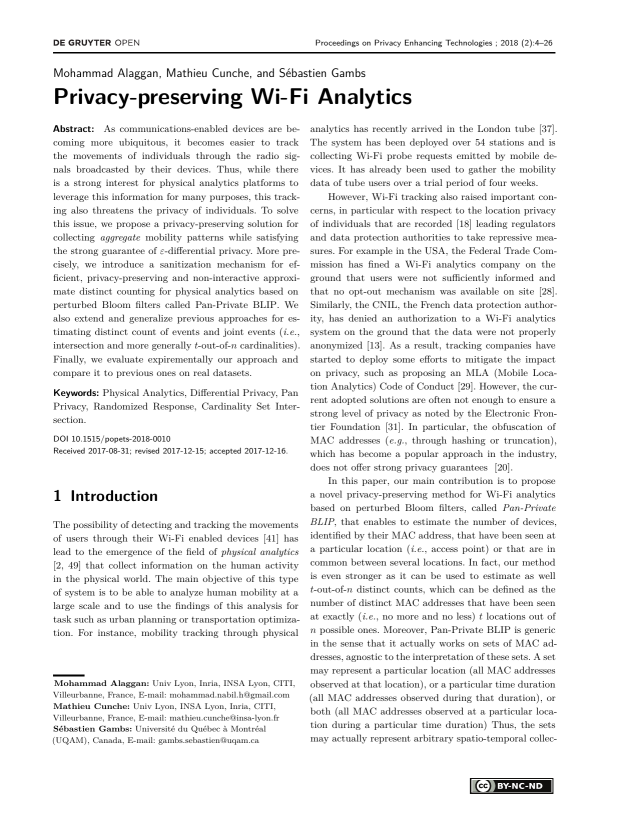