NoMoAds: Effective and Efficient Cross-App Mobile Ad-Blocking
Authors: Anastasia Shuba (University of California, Irvine), Athina Markopoulou (University of California, Irvine), Zubair Shafiq (University of Iowa)
Volume: 2018
Issue: 4
Pages: 125–140
DOI: https://doi.org/10.1515/popets-2018-0035
Abstract: Although advertising is a popular strategy for mobile app monetization, it is often desirable to block ads in order to improve usability, performance, privacy, and security. In this paper, we propose NoMoAds to block ads served by any app on a mobile device. NoMoAds leverages the network interface as a universal vantage point: it can intercept, inspect, and block outgoing packets from all apps on a mobile device. NoMoAds extracts features from packet headers and/or payload to train machine learning classifiers for detecting ad requests. To evaluate NoMoAds, we collect and label a new dataset using both EasyList and manually created rules. We show that NoMoAds is effective: it achieves an F-score of up to 97.8% and performs well when deployed in the wild. Furthermore, NoMoAds is able to detect mobile ads that are missed by EasyList (more than one-third of ads in our dataset). We also show that NoMoAds is efficient: it performs ad classification on a per-packet basis in real-time. To the best of our knowledge, NoMoAds is the first mobile ad-blocker to effectively and efficiently block ads served across all apps using a machine learning approach.
Keywords: ad-blocker; machine learning; mobile; privacy
Copyright in PoPETs articles are held by their authors. This article is published under a Creative Commons Attribution-NonCommercial-NoDerivs 3.0 license.
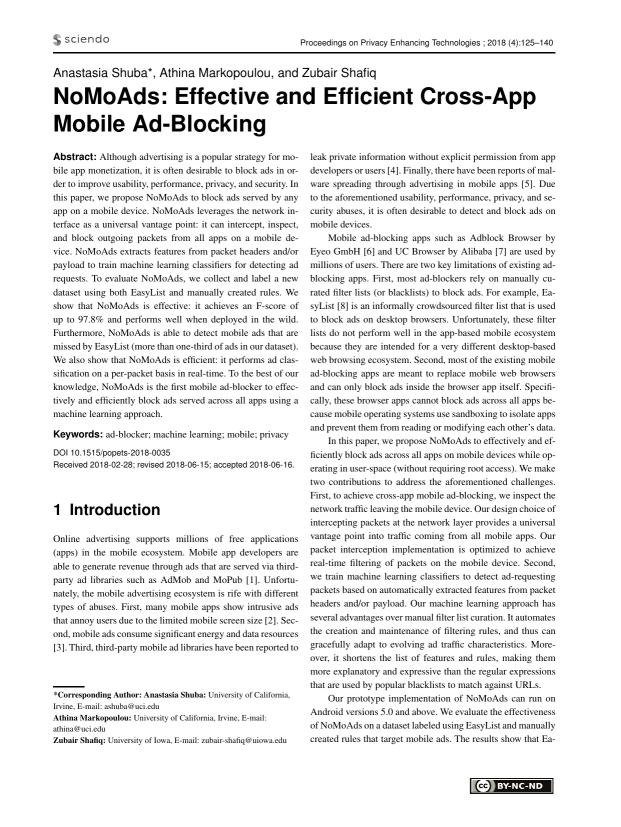