LOGAN: Membership Inference Attacks Against Generative Models
Authors: Jamie Hayes (University College London), Luca Melis (University College London), George Danezis (University College London), Emiliano De Cristofaro (University College London)
Volume: 2019
Issue: 1
Pages: 133–152
DOI: https://doi.org/10.2478/popets-2019-0008
Abstract: Generative models estimate the underlying distribution of a dataset to generate realistic samples according to that distribution. In this paper, we present the first membership inference attacks against generative models: given a data point, the adversary determines whether or not it was used to train the model. Our attacks leverage Generative Adversarial Networks (GANs), which combine a discriminative and a generative model, to detect overfitting and recognize inputs that were part of training datasets, using the discriminator’s capacity to learn statistical differences in distributions. We present attacks based on both white-box and black-box access to the target model, against several state-of-the-art generative models, over datasets of complex representations of faces (LFW), objects (CIFAR-10), and medical images (Diabetic Retinopathy). We also discuss the sensitivity of the attacks to different training parameters, and their robustness against mitigation strategies, finding that defenses are either ineffective or lead to significantly worse performances of the generative models in terms of training stability and/or sample quality.
Keywords: machine learning; privacy; inference attacks
Copyright in PoPETs articles are held by their authors. This article is published under a Creative Commons Attribution-NonCommercial-NoDerivs 3.0 license.
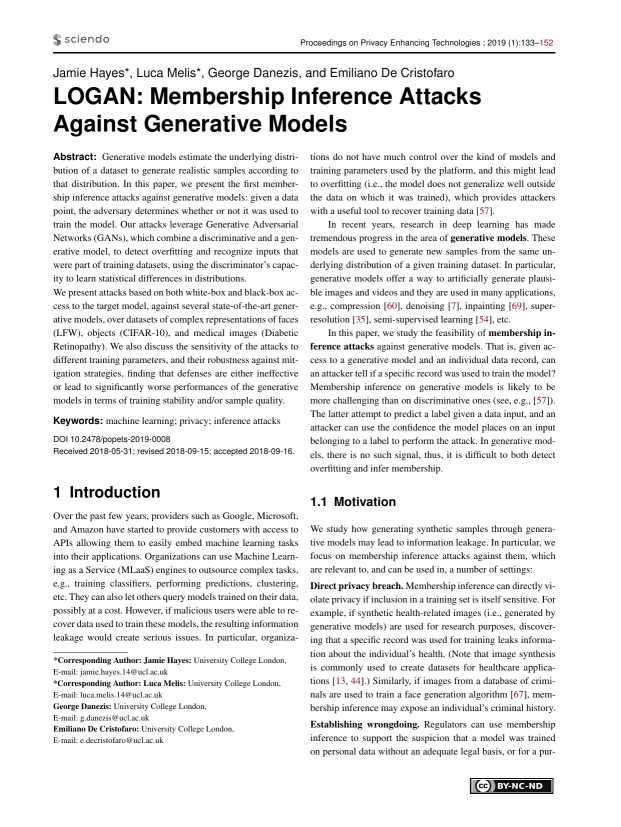