Privacy Loss Classes: The Central Limit Theorem in Differential Privacy
Authors: David M. Sommer (ETH Zurich), Sebastian Meiser (UCL), Esfandiar Mohammadi (ETH Zurich)
Volume: 2019
Issue: 2
Pages: 245–269
DOI: https://doi.org/10.2478/popets-2019-0029
Abstract: Quantifying the privacy loss of a privacypreserving mechanism on potentially sensitive data is a complex and well-researched topic; the de-facto standard for privacy measures are ε-differential privacy (DP) and its versatile relaxation (, δ)-approximate differential privacy (ADP). Recently, novel variants of (A)DP focused on giving tighter privacy bounds under continual observation. In this paper we unify many previous works via the privacy loss distribution (PLD) of a mechanism. We show that for non-adaptive mechanisms, the privacy loss under sequential composition undergoes a convolution and will converge to a Gauss distribution (the central limit theorem for DP). We derive several relevant insights: we can now characterize mechanisms by their privacy loss class, i.e., by the Gauss distribution to which their PLD converges, which allows us to give novel ADP bounds for mechanisms based on their privacy loss class; we derive exact analytical guarantees for the approximate randomized response mechanism and an exact analytical and closed formula for the Gauss mechanism, that, given ε, calculates δ, s.t., the mechanism is (ε, δ)-ADP (not an over-approximating bound).
Keywords: differential privacy, continuous observation, privacy loss, Gauss mechanism, composition
Copyright in PoPETs articles are held by their authors. This article is published under a Creative Commons Attribution-NonCommercial-NoDerivs 3.0 license.
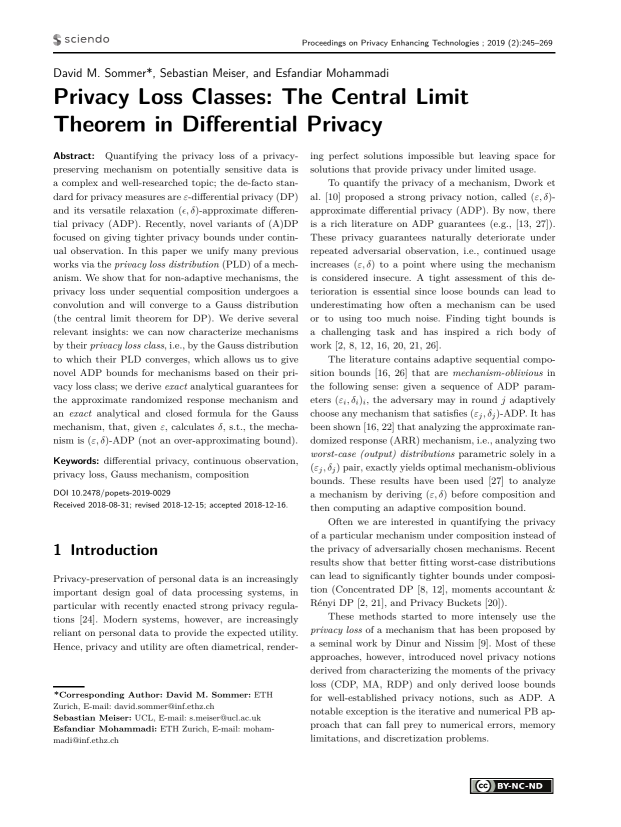