“Because... I was told... so much”: Linguistic Indicators of Mental Health Status on Twitter
Authors: Janith Weerasinghe (Affil), Kediel Morales (Affil), Rachel Greenstadt (Affil)
Volume: 2019
Issue: 4
Pages: 152–171
DOI: https://doi.org/10.2478/popets-2019-0063
Abstract: Recent studies have shown that machine learning can identify individuals with mental illnesses by analyzing their social media posts. Topics and words related to mental health are some of the top predictors. These findings have implications for early detection of mental illnesses. However, they also raise numerous privacy concerns. To fully evaluate the implications for privacy, we analyze the performance of different machine learning models in the absence of tweets that talk about mental illnesses. Our results show that machine learning can be used to make predictions even if the users do not actively talk about their mental illness. To fully understand the implications of these findings, we analyze the features that make these predictions possible. We analyze bag-of-words, word clusters, part of speech n-gram features, and topic models to understand the machine learning model and to discover language patterns that differentiate individuals with mental illnesses from a control group. This analysis confirmed some of the known language patterns and uncovered several new patterns. We then discuss the possible applications of machine learning to identify mental illnesses, the feasibility of such applications, associated privacy implications, and analyze the feasibility of potential mitigations.
Keywords: Mental Illnesses, Machine Learning, Natural Language Processing, Privacy
Copyright in PoPETs articles are held by their authors. This article is published under a Creative Commons Attribution-NonCommercial-NoDerivs 3.0 license.
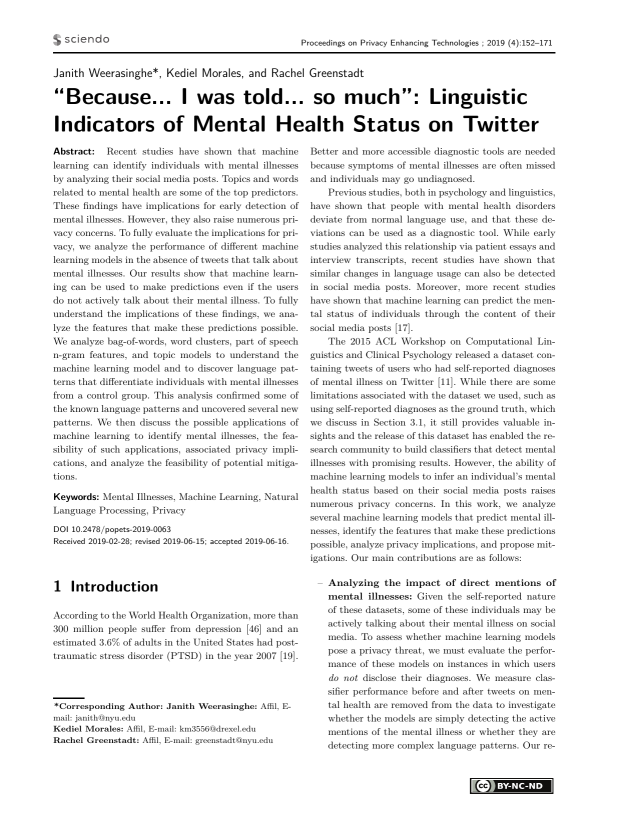