Inferring Tracker-Advertiser Relationships in the Online Advertising Ecosystem using Header Bidding
Authors: John Cook (The University of Iowa), Rishab Nithyanand (The University of Iowa), Zubair Shafiq (The University of Iowa)
Volume: 2020
Issue: 1
Pages: 65–82
DOI: https://doi.org/10.2478/popets-2020-0005
Abstract: Online advertising relies on trackers and data brokers to show targeted ads to users. To improve targeting, different entities in the intricately interwoven online advertising and tracking ecosystems are incentivized to share information with each other through client-side or server-side mechanisms. Inferring data sharing between entities, especially when it happens at the server-side, is an important and challenging research problem. In this paper, we introduce Kashf: a novel method to infer data sharing relationships between advertisers and trackers by studying how an advertiser’s bidding behavior changes as we manipulate the presence of trackers. We operationalize this insight by training an interpretable machine learning model that uses the presence of trackers as features to predict the bidding behavior of an advertiser. By analyzing the machine learning model, we can infer relationships between advertisers and trackers irrespective of whether data sharing occurs at the client-side or the server-side. We are able to identify several server-side data sharing relationships that are validated externally but are not detected by client-side cookie syncing.
Keywords: cookie syncing, header bidding, online advertising and tracking
Copyright in PoPETs articles are held by their authors. This article is published under a Creative Commons Attribution-NonCommercial-NoDerivs 3.0 license.
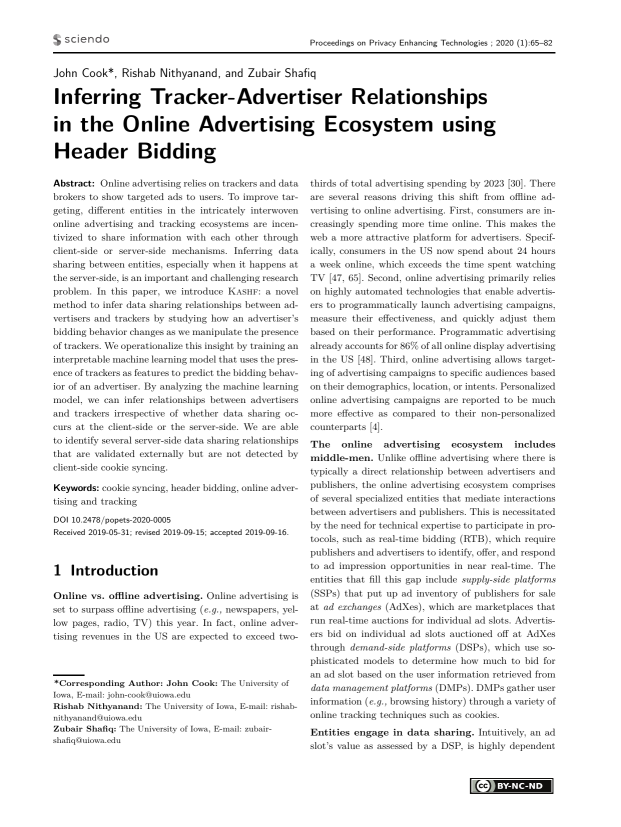