A Framework of Metrics for Differential Privacy from Local Sensitivity
Authors: Peeter Laud (Cybernetica AS), Alisa Pankova (Cybernetica AS), Martin Pettai (Cybernetica AS)
Volume: 2020
Issue: 2
Pages: 175–208
DOI: https://doi.org/10.2478/popets-2020-0023
Abstract: The meaning of differential privacy (DP) is tightly bound with the notion of distance on databases, typically defined as the number of changed rows. Considering the semantics of data, this metric may be not the most suitable one, particularly when a distance comes out as larger than the data owner desired (which would undermine privacy). In this paper, we give a mechanism to specify continuous metrics that depend on the locations and amounts of changes in a much more nuanced manner. Our metrics turn the set of databases into a Banach space. In order to construct DP information release mechanisms based on our metrics, we introduce derivative sensitivity, an analogue to local sensitivity for continuous functions. We use this notion in an analysis that determines the amount of noise to be added to the result of a database query in order to obtain a certain level of differential privacy, and demonstrate that derivative sensitivity allows us to employ powerful mechanisms from calculus to perform the analysis for a variety of queries. We have implemented the analyzer and evaluated its efficiency and precision.
Keywords: differential privacy, sensitivity
Copyright in PoPETs articles are held by their authors. This article is published under a Creative Commons Attribution-NonCommercial-NoDerivs 3.0 license.
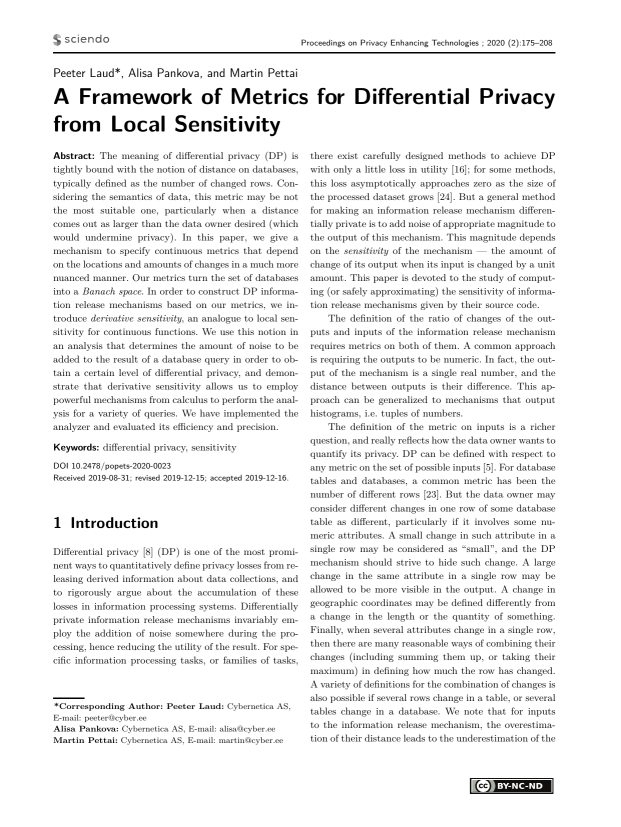