Tik-Tok: The Utility of Packet Timing in Website Fingerprinting Attacks
Authors: Mohammad Saidur Rahman (Global Cybersecurity Institute, RIT), Payap Sirinam (Navaminda Kasatriyadhiraj Royal Air Force Academy), Nate Mathews (Global Cybersecurity Institute, RIT), Kantha Girish Gangadhara (Global Cybersecurity Institute, RIT), Matthew Wright (Global Cybersecurity Institute, RIT)
Volume: 2020
Issue: 3
Pages: 5–24
DOI: https://doi.org/10.2478/popets-2020-0043
Abstract: A passive local eavesdropper can leverage Website Fingerprinting (WF) to deanonymize the web browsing activity of Tor users. The value of timing information to WF has often been discounted in recent works due to the volatility of low-level timing information. In this paper, we more carefully examine the extent to which packet timing can be used to facilitate WF attacks. We first propose a new set of timing-related features based on burst-level characteristics to further identify more ways that timing patterns could be used by classifiers to identify sites. Then we evaluate the effectiveness of both raw timing and directional timing which is a combination of raw timing and direction in a deep-learning-based WF attack. Our closed-world evaluation shows that directional timing performs best in most of the settings we explored, achieving: (i) 98.4% in undefended Tor traffic; (ii) 93.5% on WTF-PAD traffic, several points higher than when only directional information is used; and (iii) 64.7% against onion sites, 12% higher than using only direction. Further evaluations in the open-world setting show small increases in both precision (+2%) and recall (+6%) with directional-timing on WTF-PAD traffic. To further investigate the value of timing information, we perform an information leakage analysis on our proposed handcrafted features. Our results show that while timing features leak less information than directional features, the information contained in each feature is mutually exclusive to one another and can thus improve the robustness of a classifier.
Keywords: Anonymity Systems, Attack, Website Fingerprinting, Privacy, Tor;
Copyright in PoPETs articles are held by their authors. This article is published under a Creative Commons Attribution-NonCommercial-NoDerivs 3.0 license.
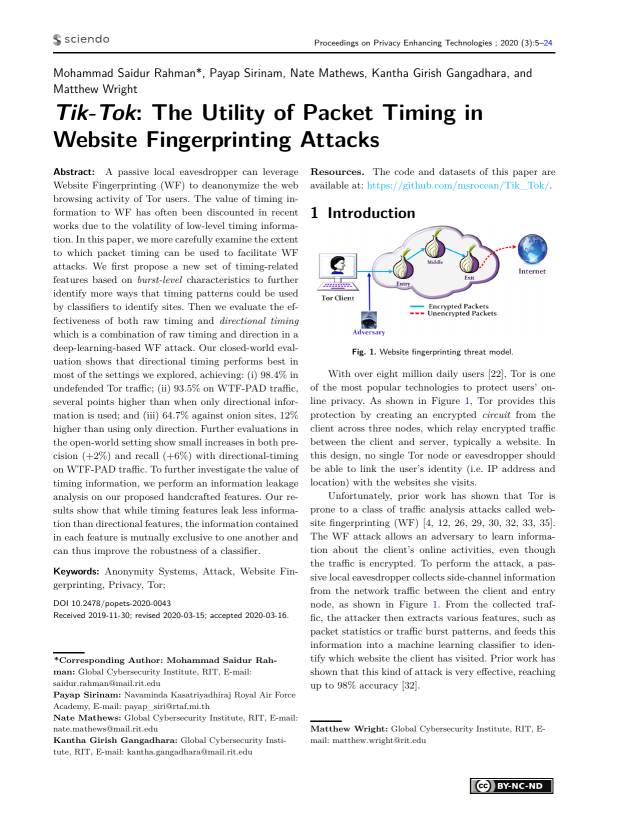