Exposing Private User Behaviors of Collaborative Filtering via Model Inversion Techniques
Authors: Seira Hidano (KDDI Research, Inc.), Takao Murakami (National Institute of Advanced Industrial Science and Technology (AIST)), Shuichi Katsumata (National Institute of Advanced Industrial Science and Technology (AIST)), Shinsaku Kiyomoto (KDDI Research, Inc.), Goichiro Hanaoka (National Institute of Advanced Industrial Science and Technology (AIST))
Volume: 2020
Issue: 3
Pages: 264–283
DOI: https://doi.org/10.2478/popets-2020-0052
Abstract: Privacy risks of collaborative filtering (CF) have been widely studied. The current state-of-theart inference attack on user behaviors (e.g., ratings/purchases on sensitive items) for CF is by Calandrino et al. (S&P, 2011). They showed that if an adversary obtained a moderate amount of user’s public behavior before some time T , she can infer user’s private behavior after time T . However, the existence of an attack that infers user’s private behavior before T remains open. In this paper, we propose the first inference attack that reveals past private user behaviors. Our attack departs from previous techniques and is based on model inversion (MI). In particular, we propose the first MI attack on factorization-based CF systems by leveraging data poisoning by Li et al. (NIPS, 2016) in a novel way. We inject malicious users into the CF system so that adversarialy chosen “decoy” items are linked with user’s private behaviors. We also show how to weaken the assumption made by Li et al. on the information available to the adversary from the whole rating matrix to only the item profile and how to create malicious ratings effectively. We validate the effectiveness of our inference algorithm using two real-world datasets.
Keywords: inference attacks, model inversion attacks, recommender systems, collaborative filtering, data poisoning, privacy exposure
Copyright in PoPETs articles are held by their authors. This article is published under a Creative Commons Attribution-NonCommercial-NoDerivs 3.0 license.
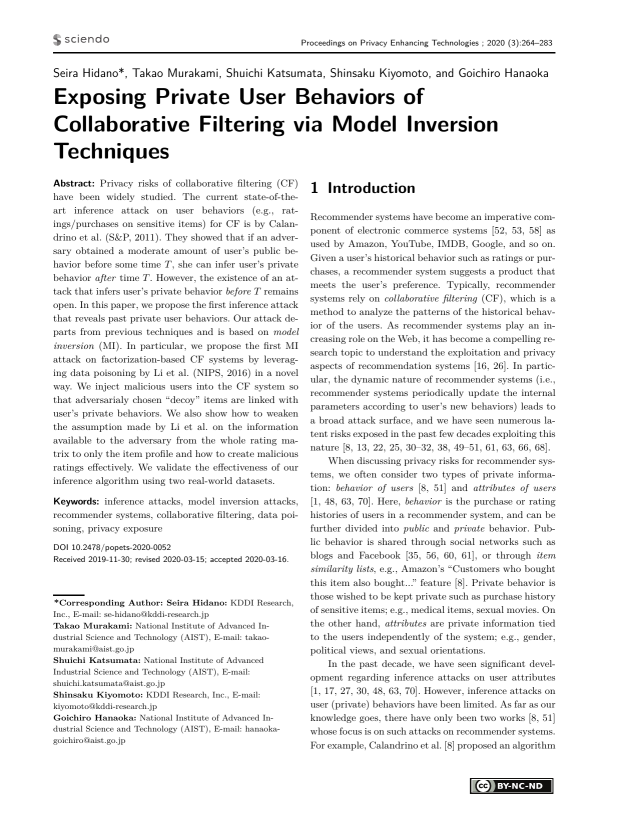