Publishing Community-Preserving Attributed Social Graphs with a Differential Privacy Guarantee
Authors: Xihui Chen (SnT, University of Luxembourg, Esch-surAlzette, Luxembourg), Sjouke Mauw (DCS, University of Luxembourg, Esch-surAlzette, Luxembourg), Yunior Ramírez-Cruz (SnT, University of Luxembourg, Esch-sur-Alzette, Luxembourg)
Volume: 2020
Issue: 4
Pages: 131–152
DOI: https://doi.org/10.2478/popets-2020-0066
Abstract: We present a novel method for publishing differentially private synthetic attributed graphs. Our method allows, for the first time, to publish synthetic graphs simultaneously preserving structural properties, user attributes and the community structure of the original graph. Our proposal relies on CAGM, a new community-preserving generative model for attributed graphs. We equip CAGM with efficient methods for attributed graph sampling and parameter estimation. For the latter, we introduce differentially private computation methods, which allow us to release communitypreserving synthetic attributed social graphs with a strong formal privacy guarantee. Through comprehensive experiments, we show that our new model outperforms its most relevant counterparts in synthesising differentially private attributed social graphs that preserve the community structure of the original graph, as well as degree sequences and clustering coefficients.
Keywords: attributed social graphs, generative models, differential privacy, community detection
Copyright in PoPETs articles are held by their authors. This article is published under a Creative Commons Attribution-NonCommercial-NoDerivs 3.0 license.
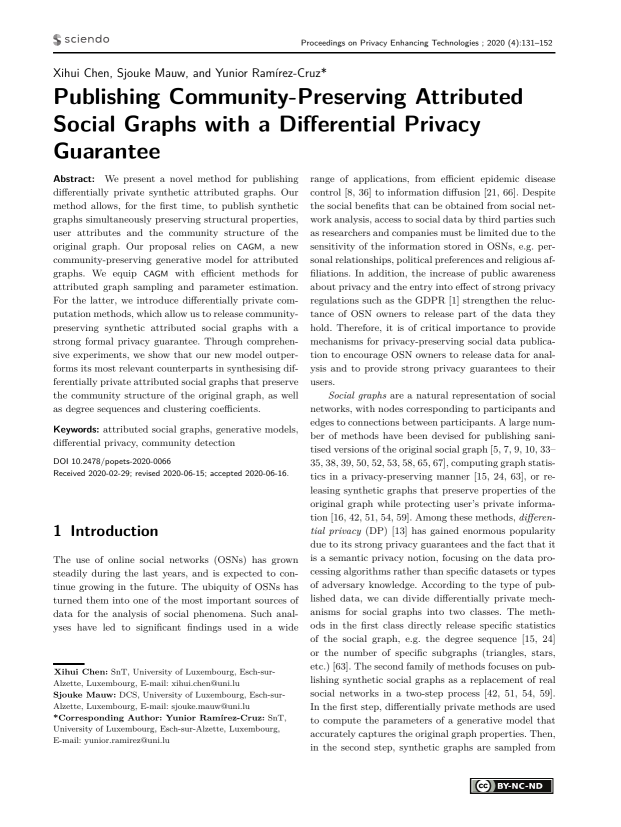