GANDaLF: GAN for Data-Limited Fingerprinting
Authors: Se Eun Oh (University of Minnesota), Nate Mathews (Rochester Institute of Technology), Mohammad Saidur Rahman (Rochester Institute of Technology), Matthew Wright (Rochester Institute of Technology), Nicholas Hopper (University of Minnesota)
Volume: 2021
Issue: 2
Pages: 305–322
DOI: https://doi.org/10.2478/popets-2021-0029
Abstract: We introduce Generative Adversarial Networks for Data-Limited Fingerprinting (GANDaLF), a new deep-learning-based technique to perform Website Fingerprinting (WF) on Tor traffic. In contrast to most earlier work on deep-learning for WF, GANDaLF is intended to work with few training samples, and achieves this goal through the use of a Generative Adversarial Network to generate a large set of “fake” data that helps to train a deep neural network in distinguishing between classes of actual training data. We evaluate GANDaLF in low-data scenarios including as few as 10 training instances per site, and in multiple settings, including fingerprinting of website index pages and fingerprinting of non-index pages within a site. GANDaLF achieves closed-world accuracy of 87% with just 20 instances per site (and 100 sites) in standard WF settings. In particular, GANDaLF can outperform Var-CNN and Triplet Fingerprinting (TF) across all settings in subpage fingerprinting. For example, GANDaLF outperforms TF by a 29% margin and Var-CNN by 38% for training sets using 20 instances per site.
Copyright in PoPETs articles are held by their authors. This article is published under a Creative Commons Attribution-NonCommercial-NoDerivs 3.0 license.
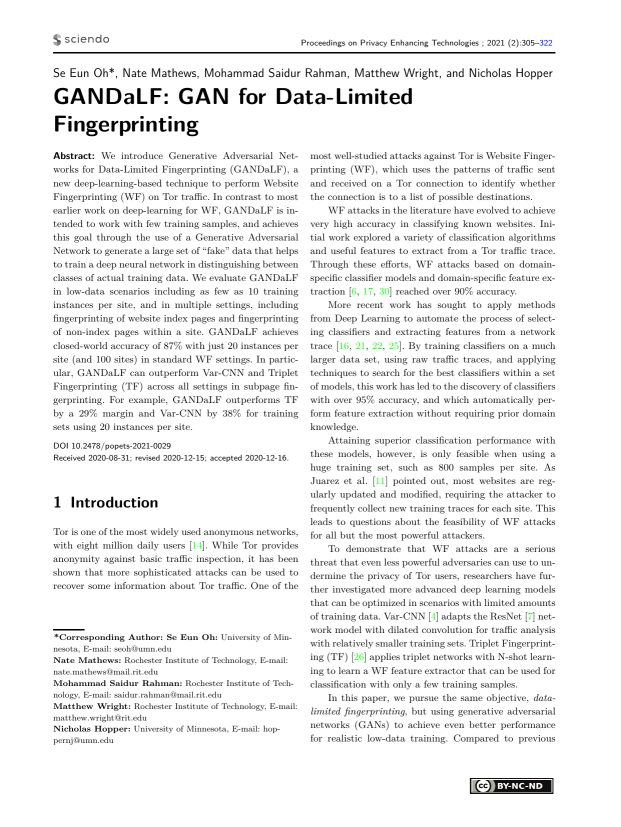