Revisiting Membership Inference Under Realistic Assumptions
Authors: Bargav Jayaraman (University of Virginia, USA), Lingxiao Wang (University of California Los Angeles, USA), Katherine Knipmeyer (University of Virginia, USA), Quanquan Gu (University of California Los Angeles, USA), David Evans (University of Virginia, USA)
Volume: 2021
Issue: 2
Pages: 348–368
DOI: https://doi.org/10.2478/popets-2021-0031
Abstract: We study membership inference in settings where assumptions commonly used in previous research are relaxed. First, we consider cases where only a small fraction of the candidate pool targeted by the adversary are members and develop a PPV-based metric suitable for this setting. This skewed prior setting is more realistic than the balanced prior setting typically considered. Second, we consider adversaries that select inference thresholds according to their attack goals, such as identifying as many members as possible with a given false positive tolerance. We develop a threshold selection designed for achieving particular attack goals. Since previous inference attacks fail in imbalanced prior settings, we develop new inference attacks based on the intuition that inputs corresponding to training set members will be near a local minimum in the loss function. An attack that combines this with thresholds on the per-instance loss can achieve high PPV even in settings where other attacks are ineffective.
Copyright in PoPETs articles are held by their authors. This article is published under a Creative Commons Attribution-NonCommercial-NoDerivs 3.0 license.
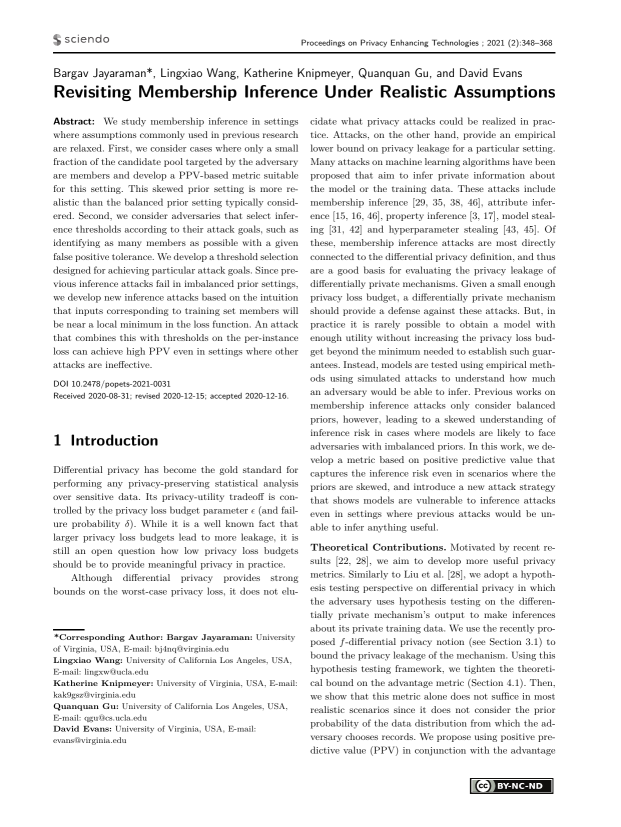