privGAN: Protecting GANs from membership inference attacks at low cost to utility
Authors: Sumit Mukherjee (AI for Good Research Lab, Microsoft, USA.), Yixi Xu (AI for Good Research Lab, Microsoft, USA.), Anusua Trivedi (AI for Good Research Lab, Microsoft, USA.), Nabajyoti Patowary (Microsoft, USA.), Juan L. Ferres (AI for Good Research Lab, Microsoft, USA.)
Volume: 2021
Issue: 3
Pages: 142–163
DOI: https://doi.org/10.2478/popets-2021-0041
Abstract: Generative Adversarial Networks (GANs) have made releasing of synthetic images a viable approach to share data without releasing the original dataset. It has been shown that such synthetic data can be used for a variety of downstream tasks such as training classifiers that would otherwise require the original dataset to be shared. However, recent work has shown that the GAN models and their synthetically generated data can be used to infer the training set membership by an adversary who has access to the entire dataset and some auxiliary information. Current approaches to mitigate this problem (such as DPGAN [1]) lead to dramatically poorer generated sample quality than the original non–private GANs. Here we develop a new GAN architecture (privGAN), where the generator is trained not only to cheat the discriminator but also to defend membership inference attacks. The new mechanism is shown to empirically provide protection against this mode of attack while leading to negligible loss in downstream performances. In addition, our algorithm has been shown to explicitly prevent memorization of the training set, which explains why our protection is so effective. The main contributions of this paper are: i) we propose a novel GAN architecture that can generate synthetic data in a privacy preserving manner with minimal hyperparameter tuning and architecture selection, ii) we provide a theoretical understanding of the optimal solution of the privGAN loss function, iii) we empirically demonstrate the effectiveness of our model against several white and black–box attacks on several benchmark datasets, iv) we empirically demonstrate on three common benchmark datasets that synthetic images generated by privGAN lead to negligible loss in downstream performance when compared against non– private GANs. While we have focused on benchmarking privGAN exclusively on image datasets, the architecture of privGAN is not exclusive to image datasets and can be easily extended to other types of datasets. Repository link: https://github.com/microsoft/privGAN.
Keywords: Membership inference, GANs
Copyright in PoPETs articles are held by their authors. This article is published under a Creative Commons Attribution-NonCommercial-NoDerivs 3.0 license.
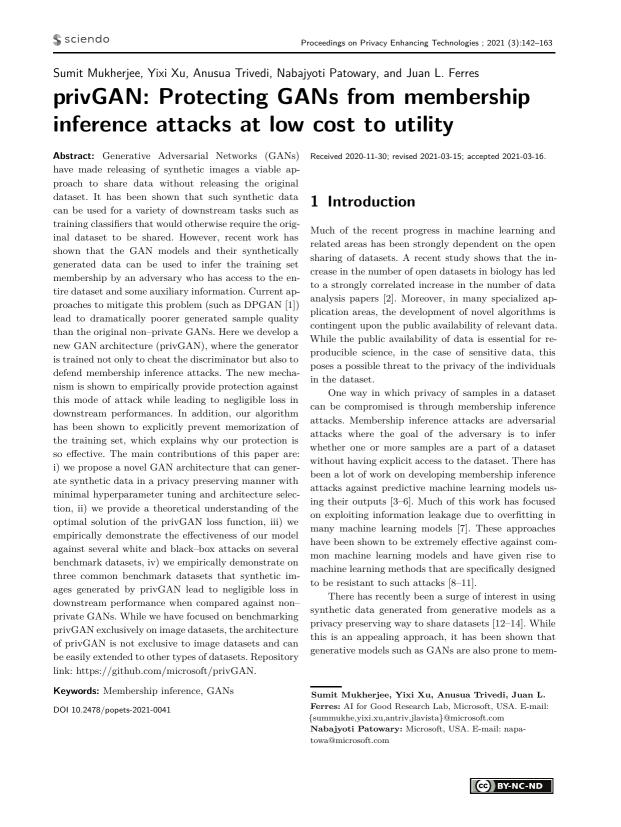