Zen and the art of model adaptation: Low-utility-cost attack mitigations in collaborative machine learning
Authors: Dmitrii Usynin (Department of Computing, Imperial College London; Department of Diagnostic and Interventional Radiology, Technical University of Munich), Daniel Rueckert (Institute for Artificial Intelligence in Medicine, Technical University of Munich; Department of Computing, Imperial College London), Jonathan Passerat-Palmbach (Department of Computing, Imperial College London; ConsenSys Health, New York, NY, USA), Georgios Kaissis (Institute for Artificial Intelligence in Medicine, Technical University of Munich; Department of Computing, Imperial College London, Germany)
Volume: 2022
Issue: 1
Pages: 274–290
DOI: https://doi.org/10.2478/popets-2022-0014
Abstract: In this study, we aim to bridge the gap between the theoretical understanding of attacks against collaborative machine learning workflows and their practical ramifications by considering the effects of model architecture, learning setting and hyperparameters on the resilience against attacks. We refer to such mitigations as model adaptation. Through extensive experimentation on both, benchmark and real-life datasets, we establish a more practical threat model for collaborative learning scenarios. In particular, we evaluate the impact of model adaptation by implementing a range of attacks belonging to the broader categories of model inversion and membership inference. Our experiments yield two noteworthy outcomes: they demonstrate the difficulty of actually conducting successful attacks under realistic settings when model adaptation is employed and they highlight the challenge inherent in successfully combining model adaptation and formal privacy-preserving techniques to retain the optimal balance between model utility and attack resilience.
Keywords: privacy, computer vision, federated learning, membership inference, model inversion
Copyright in PoPETs articles are held by their authors. This article is published under a Creative Commons Attribution-NonCommercial-NoDerivs 3.0 license.
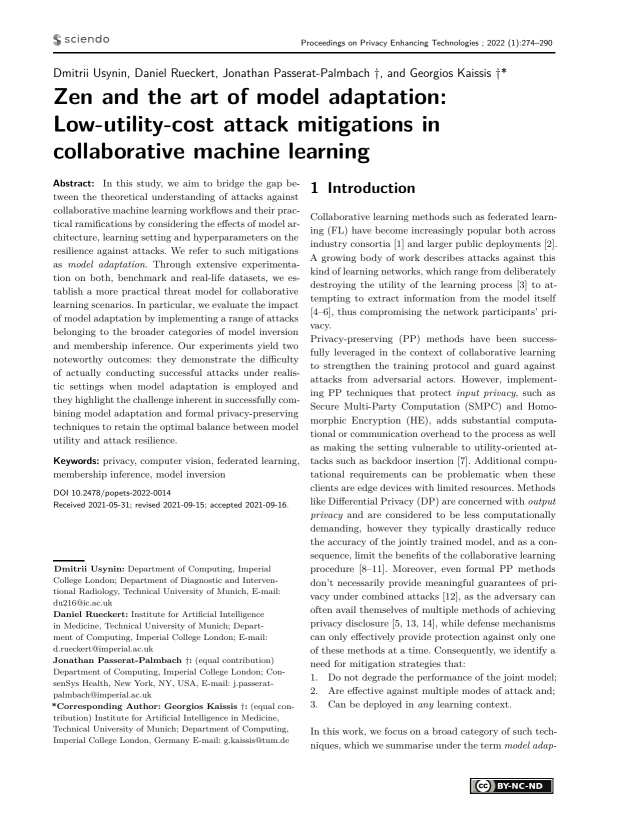