Employees’ privacy perceptions: exploring the dimensionality and antecedents of personal data sensitivity and willingness to disclose
Authors: Jan Tolsdorf (Bonn-Rhein-Sieg University of Applied Sciences), Delphine Reinhardt (University of Göttingen), Luigi Lo Iacono (Bonn-Rhein-Sieg University of Applied Sciences)
Volume: 2022
Issue: 2
Pages: 68–94
DOI: https://doi.org/10.2478/popets-2022-0036
Abstract: The processing of employees’ personal data is dramatically increasing, yet there is a lack of tools that allow employees to manage their privacy. In order to develop these tools, one needs to understand what sensitive personal data are and what factors influence employees’ willingness to disclose. Current privacy research, however, lacks such insights, as it has focused on other contexts in recent decades. To fill this research gap, we conducted a cross-sectional survey with 553 employees from Germany. Our survey provides multiple insights into the relationships between perceived data sensitivity and willingness to disclose in the employment context. Among other things, we show that the perceived sensitivity of certain types of data differs substantially from existing studies in other contexts. Moreover, currently used legal and contextual distinctions between different types of data do not accurately reflect the subtleties of employees’ perceptions. Instead, using 62 different data elements, we identified four groups of personal data that better reflect the multi-dimensionality of perceptions. However, previously found common disclosure antecedents in the context of online privacy do not seem to affect them. We further identified three groups of employees that differ in their perceived data sensitivity and willingness to disclose, but neither in their privacy beliefs nor in their demographics. Our findings thus provide employers, policy makers, and researchers with a better understanding of employees’ privacy perceptions and serve as a basis for future targeted research on specific types of personal data and employees.
Keywords: employee privacy, factor analysis, structural equation modeling, latent class analysis
Copyright in PoPETs articles are held by their authors. This article is published under a Creative Commons Attribution-NonCommercial-NoDerivs 3.0 license.
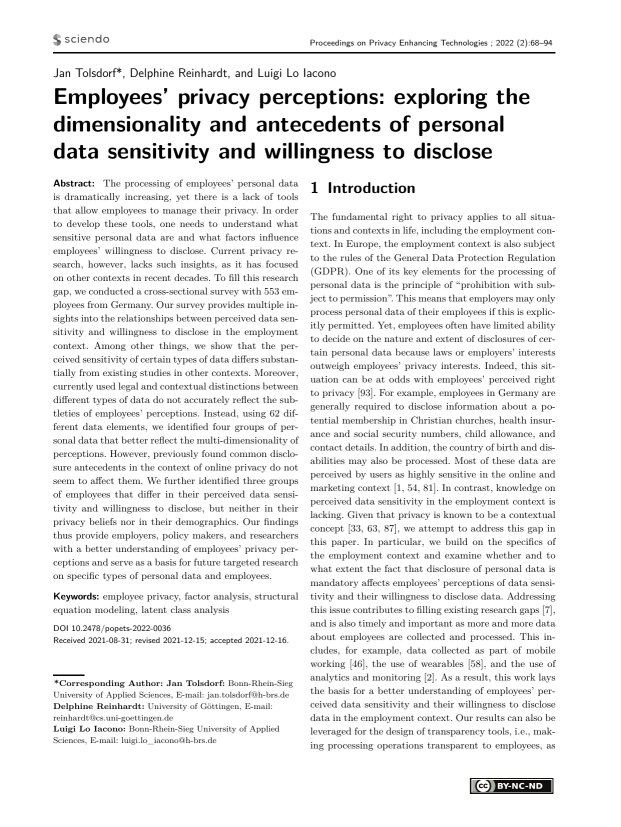