Knowledge Cross-Distillation for Membership Privacy
Authors: Rishav Chourasia (National University of Singapore), Batnyam Enkhtaivan (NEC Corporation), Kunihiro Ito (NEC Corporation), Junki Mori (NEC Corporation), Isamu Teranishi (NEC Corporation), Hikaru Tsuchida (NEC Corporation)
Volume: 2022
Issue: 2
Pages: 362–377
DOI: https://doi.org/10.2478/popets-2022-0050
Abstract: A membership inference attack (MIA) poses privacy risks for the training data of a machine learning model. With an MIA, an attacker guesses if the target data are a member of the training dataset. The state-ofthe-art defense against MIAs, distillation for membership privacy (DMP), requires not only private data for protection but a large amount of unlabeled public data. However, in certain privacy-sensitive domains, such as medicine and finance, the availability of public data is not guaranteed. Moreover, a trivial method for generating public data by using generative adversarial networks significantly decreases the model accuracy, as reported by the authors of DMP. To overcome this problem, we propose a novel defense against MIAs that uses knowledge distillation without requiring public data. Our experiments show that the privacy protection and accuracy of our defense are comparable to those of DMP for the benchmark tabular datasets used in MIA research, Purchase100 and Texas100, and our defense has a much better privacy-utility trade-off than those of the existing defenses that also do not use public data for the image dataset CIFAR10.
Keywords: privacy-preserving machine learning, membership inference attacks, knowledge distillation
Copyright in PoPETs articles are held by their authors. This article is published under a Creative Commons Attribution-NonCommercial-NoDerivs 3.0 license.
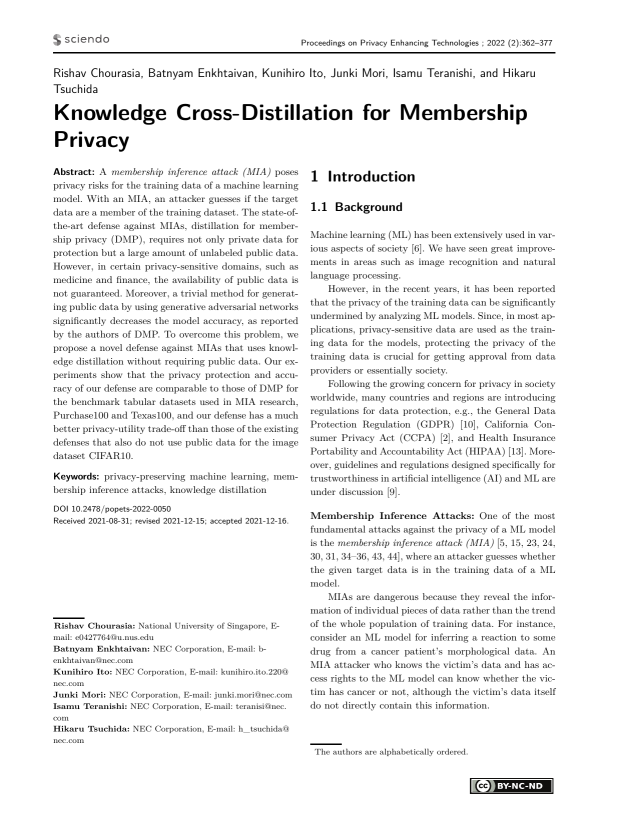