Adversarial Images Against Super-Resolution Convolutional Neural Networks for Free
Authors: Arezoo Rajabi (University of Washington), Mahdieh Abbasi (Universite Laval), Rakesh B. Bobba (Oregon State University), Kimia Tajik (Case Western Reserve University)
Volume: 2022
Issue: 3
Pages: 120–139
DOI: https://doi.org/10.56553/popets-2022-0065
Abstract: Super-Resolution Convolutional Neural Networks (SRCNNs) with their ability to generate highresolution images from low-resolution counterparts, exacerbate the privacy concerns emerging from automated Convolutional Neural Networks (CNNs)-based image classifiers. In this work, we hypothesize and empirically show that adversarial examples learned over CNN image classifiers can survive processing by SRCNNs and lead them to generate poor quality images that are hard to classify correctly. We demonstrate that a user with a small CNN is able to learn adversarial noise without requiring any customization for SRCNNs and thwart the privacy threat posed by a pipeline of SRCNN and CNN classifiers (95.8% fooling rate for Fast Gradient Sign with ε = 0.03). We evaluate the survivability of adversarial images generated in both black-box and white-box settings and show that black-box adversarial learning (when both CNN classifier and SRCNN are unknown) is at least as effective as white-box adversarial learning (when only CNN classifier is known). We also assess our hypothesis on adversarial robust CNNs and observe that the supper-resolved white-box adversarial examples can fool these CNNs more than 71.5% of the time.
Keywords: Survivability of Adversarial Example, SuperResolution Convolutional Neural networks, Image Privacy
Copyright in PoPETs articles are held by their authors. This article is published under a Creative Commons Attribution-NonCommercial-NoDerivs 3.0 license.
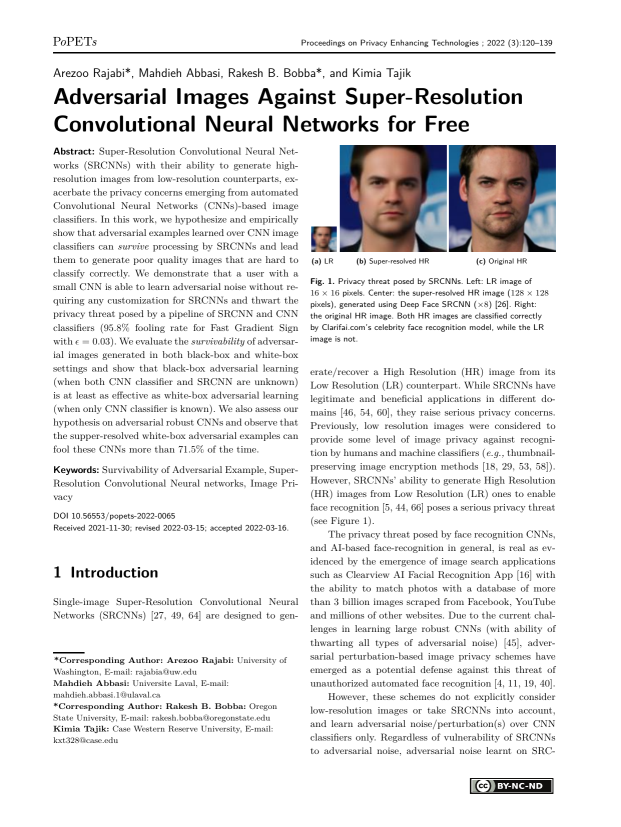