Trace Oddity: Methodologies for Data-Driven Traffic Analysis on Tor
Authors: Vera Rimmer (imec-DistriNet, KU Leuven), Theodor Schnitzler (Ruhr-Universität Bochum), Tom Van Goethem (imec-DistriNet, KU Leuven), Abel Rodríguez Romero (imec-DistriNet, KU Leuven), Wouter Joosen (imec-DistriNet, KU Leuven), Katharina Kohls (Radboud University)
Volume: 2022
Issue: 3
Pages: 314–335
DOI: https://doi.org/10.56553/popets-2022-0074
Abstract: Traffic analysis attacks against encrypted web traffic are a persisting problem. However, there is a large gap between the scientific estimate of attack threats and the real-world situation. As traffic analysis attacks depend on very specific metadata information, they are sensitive to artificial changes in the transmission characteristics. While the advent of deep learning greatly improves the performance rates of traffic analysis attacks on Tor in research settings, deep neural networks are known for being implicitly vulnerable to artifacts in data. Removing artifacts from our experimental setups is essential to minimizing the risk of evaluation bias. In this work, we study a state-of-the-art end-to-end traffic correlation attack on Tor and propose a novel data collection setup. Our design addresses the key constraint of prior work: instead of using a single proxy node for collecting exit traffic, we deploy multiple proxies. Our extensive analysis shows that in the multi-proxy design (i) end-to-end round-trip times are more realistic than in the original design, and that (ii) traffic correlation attack performance degrades significantly on realistic timings. For a reliable and informative evaluation, we develop a general scientific methodology for replication and comparison of machine and deep-learning attacks on Tor. Our evaluation indicates high relevance of the multi-proxy data collection setup and the novel dataset.
Keywords: end-to-end correlation attack, traffic analysis, data collection, anonymity, deep learning.
Copyright in PoPETs articles are held by their authors. This article is published under a Creative Commons Attribution-NonCommercial-NoDerivs 3.0 license.
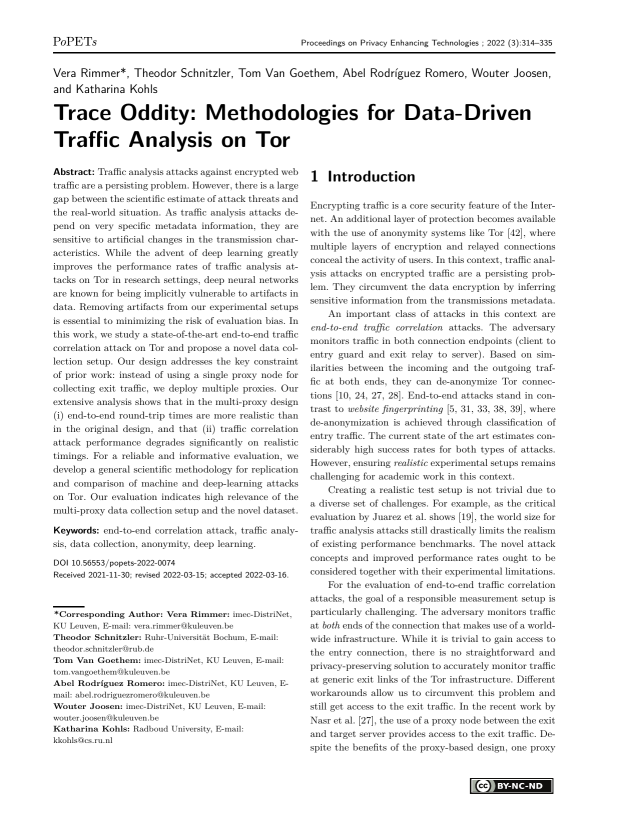