Towards Sparse Federated Analytics: Location Heatmaps under Distributed Differential Privacy with Secure Aggregation
Authors: Eugene Bagdasaryan (Cornell Tech), Peter Kairouz (Google), Stefan Mellem (Google), Adrià Gascón (Google), Kallista Bonawitz (Google), Deborah Estrin (Cornell Tech), Marco Gruteser (Google)
Volume: 2022
Issue: 4
Pages: 162–182
DOI: https://doi.org/10.56553/popets-2022-0104
Abstract: We design a scalable algorithm to privately generate location heatmaps over decentralized data from millions of user devices. It aims to ensure differential privacy before data becomes visible to a service provider while maintaining high data accuracy and minimizing resource consumption on users’ devices. To achieve this, we revisit distributed differential privacy based on recent results in secure multiparty computation, and we design a scalable and adaptive distributed differential privacy approach for location analytics. Evaluation on public location datasets shows that this approach successfully generates metropolitan-scale heatmaps from millions of user samples with a worstcase client communication overhead that is significantly smaller than existing state-of-the-art private protocols of similar accuracy.
Keywords: federated analytics, location privacy, differential privacy, secure aggregation
Copyright in PoPETs articles are held by their authors. This article is published under a Creative Commons Attribution-NonCommercial-NoDerivs 3.0 license.
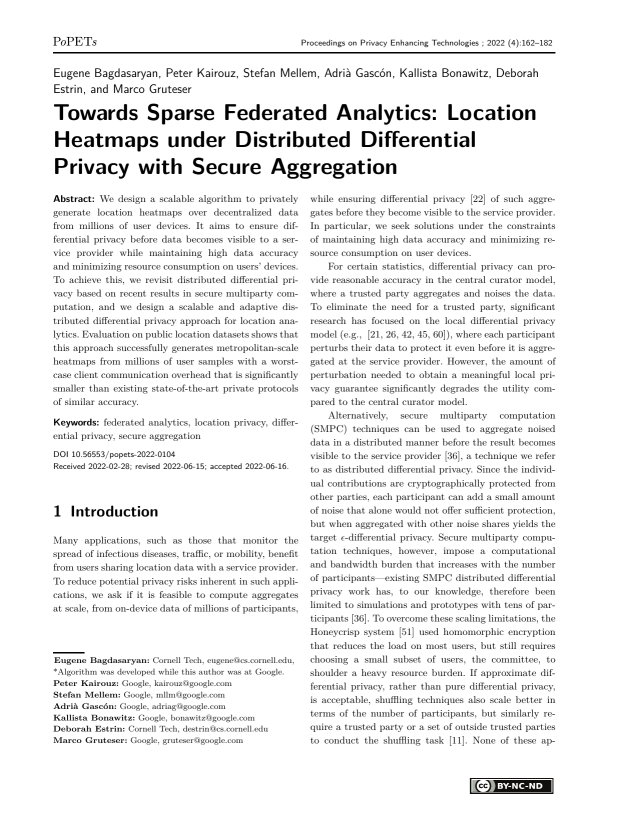