Differentially Private Speaker Anonymization
Authors: Ali Shahin Shamsabadi (The Alan Turing Institute, Vector Institute, Université de Lille, Inria, CNRS, Centrale Lille, UMR 9189 - CRIStAL, F-59000 Lille, France), Brij Mohan Lal Srivastava (Université de Lille, Inria, CNRS, Centrale Lille, UMR 9189 - CRIStAL, F-59000 Lille, France), Aurélien Bellet (Université de Lille, Inria, CNRS, Centrale Lille, UMR 9189 - CRIStAL, F-59000 Lille, France), Nathalie Vauquier (Université de Lille, Inria, CNRS, Centrale Lille, UMR 9189 - CRIStAL, F-59000 Lille, France), Emmanuel Vincent (Université de Lorraine, CNRS, Inria, LORIA, F-54000 Nancy, France), Mohamed Maouche (Université de Lille, Inria, CNRS, Centrale Lille, UMR 9189 - CRIStAL, F-59000 Lille, France), Marc Tommasi (Université de Lille, Inria, CNRS, Centrale Lille, UMR 9189 - CRIStAL, F-59000 Lille, France), Nicolas Papernot (Vector Institute, University of Toronto)
Volume: 2023
Issue: 1
Pages: 98–114
DOI: https://doi.org/10.56553/popets-2023-0007
Abstract: Sharing real-world speech utterances is key to the training and deployment of voice-based services. However, it also raises privacy risks as speech contains a wealth of personal data. Speaker anonymization aims to remove speaker information from a speech utterance while leaving its linguistic and prosodic attributes intact. State-of-the-art techniques operate by disentangling the speaker information (represented via a speaker embedding) from these attributes and re-synthesizing speech based on the speaker embedding of another speaker. Prior research in the privacy community has shown that anonymization often provides brittle privacy protection, even less so any provable guarantee. In this work, we show that disentanglement is indeed not perfect: linguistic and prosodic attributes still contain speaker information. We remove speaker information from these attributes by introducing differentially private feature extractors based on an autoencoder and an automatic speech recognizer, respectively, trained using noise layers. We plug these extractors in the state-of-the-art anonymization pipeline and generate, for the first time, private speech utterances with a provable upper bound on the speaker information they contain. We evaluate empirically the privacy and utility resulting from our differentially private speaker anonymization approach on the LibriSpeech data set. Experimental results show that the generated utterances retain very high utility for automatic speech recognition training and inference, while being much better protected against strong adversaries who leverage the full knowledge of the anonymization process to try to infer the speaker identity.
Keywords: speaker anonymization, differential privacy, automatic speech recognition, automatic speaker recognition, voice-based services, privacy
Copyright in PoPETs articles are held by their authors. This article is published under a Creative Commons Attribution 4.0 license.
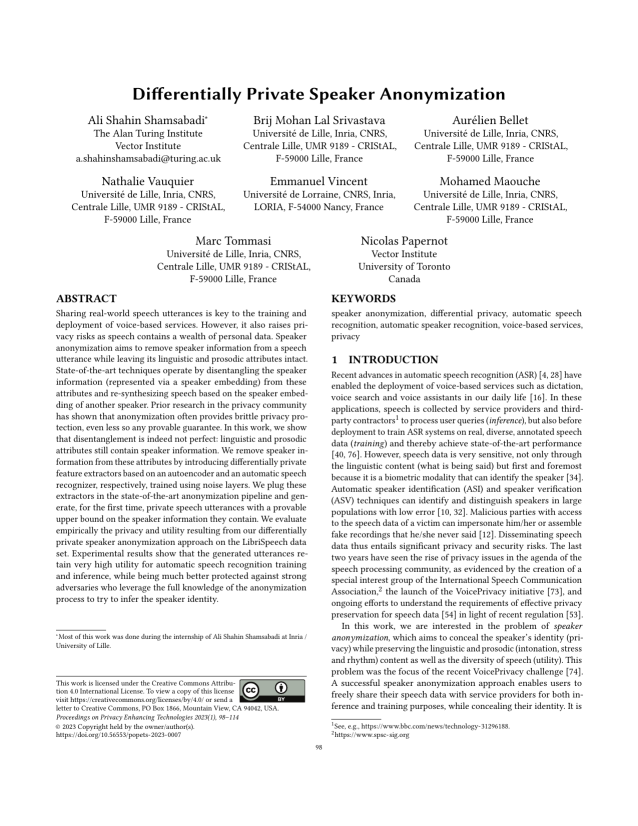