Exploring Model Inversion Attacks in the Black-box Setting
Authors: Antreas Dionysiou (University of Cyprus), Vassilis Vassiliades (CYENS Centre of Excellence), Elias Athanasopoulos (University of Cyprus)
Volume: 2023
Issue: 1
Pages: 190–206
DOI: https://doi.org/10.56553/popets-2023-0012
Abstract: Model Inversion (MI) attacks, that aim to recover semantically meaningful reconstructions for each target class, have been extensively studied and demonstrated to be successful in the white-box setting. On the other hand, black-box MI attacks demonstrate low performance in terms of both effectiveness, i.e., reconstructing samples which are identifiable as their ground-truth, and efficiency, i.e., time or queries required for completing the attack process. Whether or not effective and efficient black-box MI attacks can be conducted on complex targets, such as Convolutional Neural Networks (CNNs), currently remains unclear. In this paper, we present a feasibility study in regards to the effectiveness and efficiency of MI attacks in the black-box setting. In this context, we introduce Deep-BMI (Deep Black-box Model Inversion), a framework that supports various black-box optimizers for conducting MI attacks on deep CNNs used for image recognition. Deep-BMI’s most efficient optimizer is based on an adaptive hill climbing algorithm, whereas its most effective optimizer is based on an evolutionary algorithm capable of performing an all-class attack and returning a diversity of images in a single run. For assessing the severity of this threat, we utilize all three evaluation approaches found in the literature. In particular, we (a) conduct a user study with human participants, (b) demonstrate our actual reconstructions along with their ground-truth, and (c) use relevant quantitative metrics. Surprisingly, our results suggest that black-box MI attacks, and for complex models, are comparable, in some cases, to those reported so far in the white-box setting.
Keywords: model inversion, inference attack, security, privacy
Copyright in PoPETs articles are held by their authors. This article is published under a Creative Commons Attribution 4.0 license.
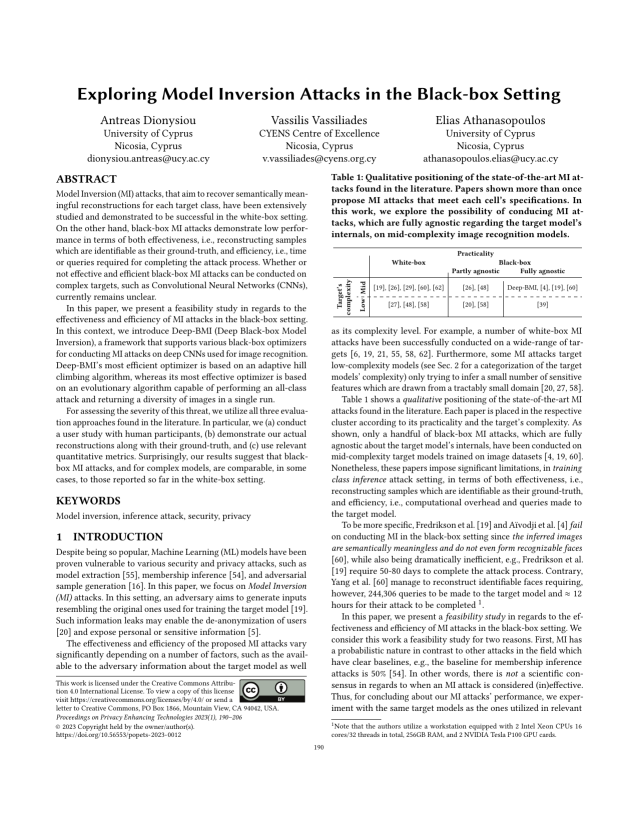