Efficient decision tree training with new data structure for secure multi-party computation
Authors: Koki Hamada (NTT Social Informatics Laboratories), Dai Ikarashi (NTT Social Informatics Laboratories), Ryo Kikuchi (NTT Social Informatics Laboratories), Koji Chida (Gunma University)
Volume: 2023
Issue: 1
Pages: 343–364
DOI: https://doi.org/10.56553/popets-2023-0021
Abstract: We propose a secure multi-party computation (MPC) protocol that constructs a secret-shared decision tree for a given secret-shared dataset. The previous MPC-based decision tree training protocol (Abspoel et al. 2021) requires $O(2^hmn log n)$ comparisons, being exponential in the tree height $h$ and with $n$ and $m$ being the number of rows and that of attributes in the dataset, respectively. The cause of the exponential number of comparisons in $h$ is that the decision tree training algorithm is based on the divide-and-conquer paradigm, where rows are padded after each split in order to hide the number of rows in the dataset. We resolve this issue via secure data structure that enables us to compute an aggregate value for every group while hiding the grouping information. By using this data structure, we can train a decision tree without padding to rows while hiding the size of the intermediate data. We specifically describes a decision tree training protocol that requires only $O(hmn log n)$ comparisons when the input attributes are continuous and the output attribute is binary. Note that the order is now linear in the tree height $h$. To demonstrate the practicality of our protocol, we implement it in an MPC framework based on a three-party secret sharing scheme. Our implementation results show that our protocol trains a decision tree with a height of 4 in 404 seconds for a dataset of $2^{20}$ rows and 11 attributes.
Keywords: secure multi-party computation, decision tree training
Copyright in PoPETs articles are held by their authors. This article is published under a Creative Commons Attribution 4.0 license.
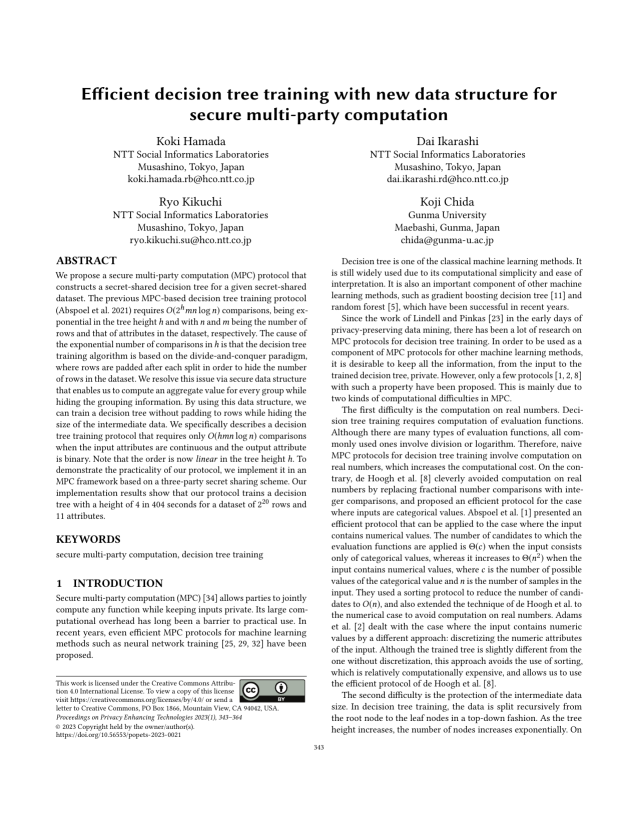