Lessons Learned: Surveying the Practicality of Differential Privacy in the Industry
Authors: Gonzalo Munilla Garrido (TUM, BMW Group), Xiaoyuan Liu (UC Berkeley), Floria Matthes (TUM), Dawn Song (UC Berkeley)
Volume: 2023
Issue: 2
Pages: 151–170
DOI: https://doi.org/10.56553/popets-2023-0045
Abstract: Since its introduction in 2006, differential privacy has emerged as a predominant statistical tool for quantifying data privacy in academic works. Yet despite the plethora of research and open-source utilities that have accompanied its rise, with limited exceptions, differential privacy has failed to achieve widespread adoption in the enterprise domain. Our study aims to shed light on the fundamental causes underlying this academic-industrial utilization gap through detailed interviews of 24 privacy practitioners across 9 major companies. We analyze the results of our survey to provide key findings and suggestions for companies striving to improve privacy protection in their data workflows and highlight the necessary and missing requirements of existing differential privacy tools, with the goal of guiding researchers working towards the broader adoption of differential privacy. Our findings indicate that analysts suffer from lengthy bureaucratic processes for requesting access to sensitive data, yet once granted, only scarcely-enforced privacy policies stand between rogue practitioners and misuse of private information. We thus argue that differential privacy can significantly improve the processes of requesting and conducting data exploration across silos, and conclude that with a few of the improvements suggested herein, the practical use of differential privacy across the enterprise is within striking distance.
Keywords: Privacy-enhancing technology, data sharing, data analytics, platform, SQL, machine learning, case study, interviews, survey
Copyright in PoPETs articles are held by their authors. This article is published under a Creative Commons Attribution 4.0 license.
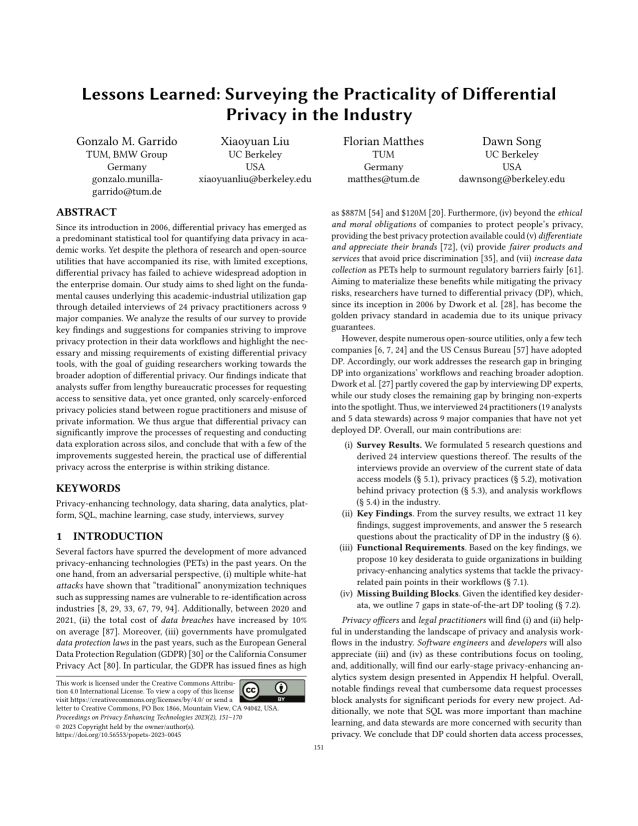