PubSub-ML: A Model Streaming Alternative to Federated Learning
Authors: Lovedeep Gondara (Simon Fraser University), Ke Wang (Simon Fraser University)
Volume: 2023
Issue: 2
Pages: 464–479
DOI: https://doi.org/10.56553/popets-2023-0063
Abstract: Federated learning is a decentralized learning framework where participating sites are engaged in a tight collaboration, forcing them into symmetric sharing and the agreement in terms of data samples, feature spaces, model types and architectures, privacy settings, and training processes. We propose PubSub-ML, Publish-Subscribe for Machine Learning, as a solution in a loose collaboration setting where each site maintains local autonomy on these decisions. In PubSub-ML, each site is either a publisher or a subscriber or both. The publishers publish differentially private machine learning models and the subscribers subscribe to published models in order to construct customized models for local use, essentially benefiting from other sites' data by distilling knowledge from publishers' models while respecting data privacy. The term “model streaming” comes from the extension of PubSub-ML to decentralized data streams with concept drift. Our extensive empirical evaluation shows that PubSub-ML outperforms federated learning methods by a significant margin.
Keywords: decentralized learning, differential privacy, federated learning
Copyright in PoPETs articles are held by their authors. This article is published under a Creative Commons Attribution 4.0 license.
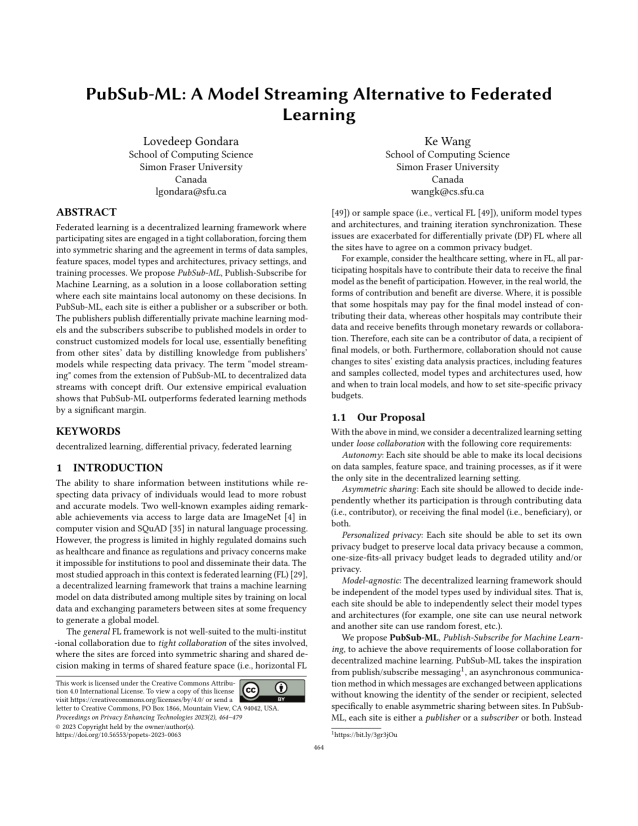