Evolution of Composition, Readability, and Structure of Privacy Policies over Two Decades
Authors: Andrick Adhikari (University of Denver), Sanchari Das (University of Denver), Rinku Dewri (University of Denver)
Volume: 2023
Issue: 3
Pages: 138–153
DOI: https://doi.org/10.56553/popets-2023-0074
Abstract: Privacy policies outline data collection and sharing practices followed by an organization, together with choice and control measures available to users to manage the process. However, users have often needed help reading and understanding such documents, regardless of their being written in a natural language. The fundamental problems with privacy policies persist despite advancements in privacy design, frameworks, and regulations. To identify the causes of privacy policies being persistently challenging to comprehend, it is vital to investigate historical policy patterns and understand the evolution of privacy policies concerning information packaging and presentation. To this aid, we create a sentence-level classifier to conduct a large-scale longitudinal analysis on different privacy policies from 130,604 organizations, totaling approximately one million policies from 1997 to 2019. We annotate 10,717 sentences from 115 policies in the OPP-115 corpus to implement the classifier and then use those annotations to train the XLNet and BERT classifiers. Results from our analysis reveal that specific data practice categories experience more frequent policy changes than others, making it challenging to track relevant information over time. In addition, we discover that every category has distinct composition, readability, and structural issues, which exacerbate when categories frequently co-occur in a document. Based on our observations, we provide recommendations for policy articulation and revision to make privacy policy documents conform to better coherence and structure.
Keywords: Sentence Classification, Longitudinal Analysis, Neural Networks, Privacy Policies, Information Organization, Change Detection
Copyright in PoPETs articles are held by their authors. This article is published under a Creative Commons Attribution 4.0 license.
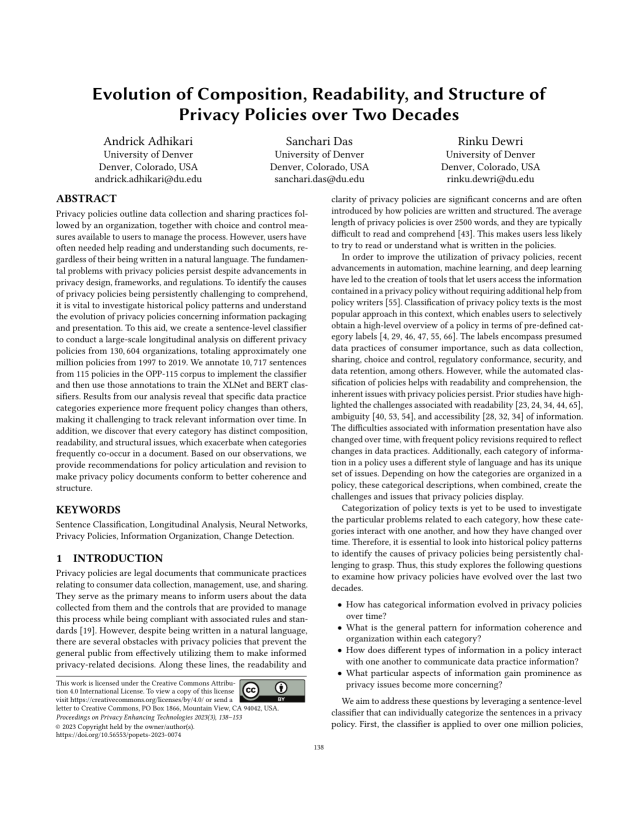