Data-Explainable Website Fingerprinting with Network Simulation
Authors: Rob Jansen (U.S. Naval Research Laboratory), Ryan Wails (U.S. Naval Research Laboratory, Georgetown University)
Volume: 2023
Issue: 4
Pages: 559–577
DOI: https://doi.org/10.56553/popets-2023-0125
Abstract: Website fingerprinting (WF) attacks allow an adversary to associate a website with the encrypted traffic patterns produced when accessing it, thus threatening to destroy the client-server unlinkability promised by anonymous communication networks. Explainable WF is an open problem in which we need to improve our understanding of (1) the machine learning models used to conduct WF attacks; and (2) the WF datasets used as inputs to those models. This paper focuses on explainable datasets; that is, we develop an alternative to the standard practice of gathering low-quality WF datasets using synthetic browsers in large networks without controlling for natural network variability. In particular, we demonstrate how network simulation can be used to produce explainable WF datasets by leveraging the simulator's high degree of control over network operation. Through a detailed investigation of the effect of network variability on WF performance, we find that: (1) training and testing WF attacks in networks with distinct levels of congestion increases the false-positive rate by as much as 200%; (2) augmenting the WF attacks by training them across several networks with varying degrees of congestion decreases the false-positive rate by as much as 83%; and (3) WF classifiers trained on completely simulated data can achieve greater than 80% accuracy when applied to the real world.
Keywords: Tor, website fingerprinting, network simulation
Copyright in PoPETs articles are held by their authors. This article is published under a Creative Commons Attribution 4.0 license.
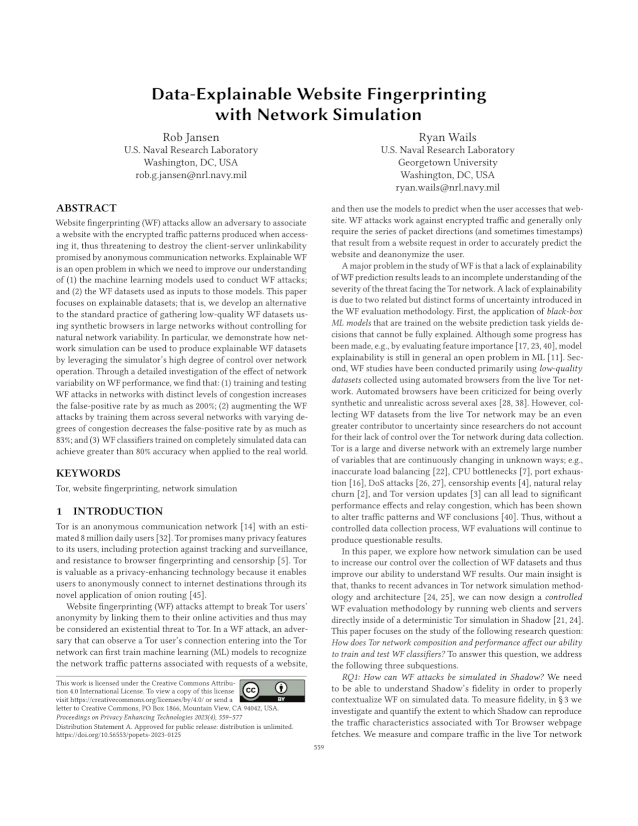