A Cautionary Tale: On the Role of Reference Data in Empirical Privacy Defenses
Authors: Caelin Kaplan (SAP Labs France, Inria, Université Côte d’Azur), Chuan Xu (Inria, Université Côte d’Azur, CNRS, I3S), Othmane Marfoq (Inria, Université Côte d’Azur, Accenture Labs), Giovanni Neglia (Inria, Université Côte d’Azur), Anderson Santana de Oliveira (SAP Labs France)
Volume: 2024
Issue: 1
Pages: 525–548
DOI: https://doi.org/10.56553/popets-2024-0031
Abstract: Within the realm of privacy-preserving machine learning, empirical privacy defenses have been proposed as a solution to achieve satisfactory levels of training data privacy without a significant drop in model utility. Most existing defenses against membership inference attacks assume access to reference data, defined as an additional dataset coming from the same (or a similar) underlying distribution as training data. Despite the common use of reference data, previous works are notably reticent about defining and evaluating reference data privacy. As gains in model utility and/or training data privacy may come at the expense of reference data privacy, it is essential that all three aspects are duly considered. In this paper, we conduct the first comprehensive analysis of empirical privacy defenses. First, we examine the availability of reference data and its privacy treatment in previous works and demonstrate its necessity for fairly comparing defenses. Second, we propose a baseline defense that enables the utility-privacy tradeoff with respect to both training and reference data to be easily understood. Our method is formulated as an empirical risk minimization with a constraint on the generalization error, which, in practice, can be evaluated as a weighted empirical risk minimization (WERM) over the training and reference datasets. Although we conceived of WERM as a simple baseline, our experiments show that, surprisingly, it outperforms the most well-studied and current state-of-the-art empirical privacy defenses using reference data for nearly all relative privacy levels of reference and training data. Our investigation also reveals that these existing methods are unable to trade off reference data privacy for model utility and/or training data privacy, and thus fail to operate outside of the high reference data privacy case. Overall, our work highlights the need for a proper evaluation of the triad model utility / training data privacy / reference data privacy when comparing privacy defenses.
Keywords: privacy-preserving machine learning, empirical privacy defenses, statistical learning
Copyright in PoPETs articles are held by their authors. This article is published under a Creative Commons Attribution 4.0 license.
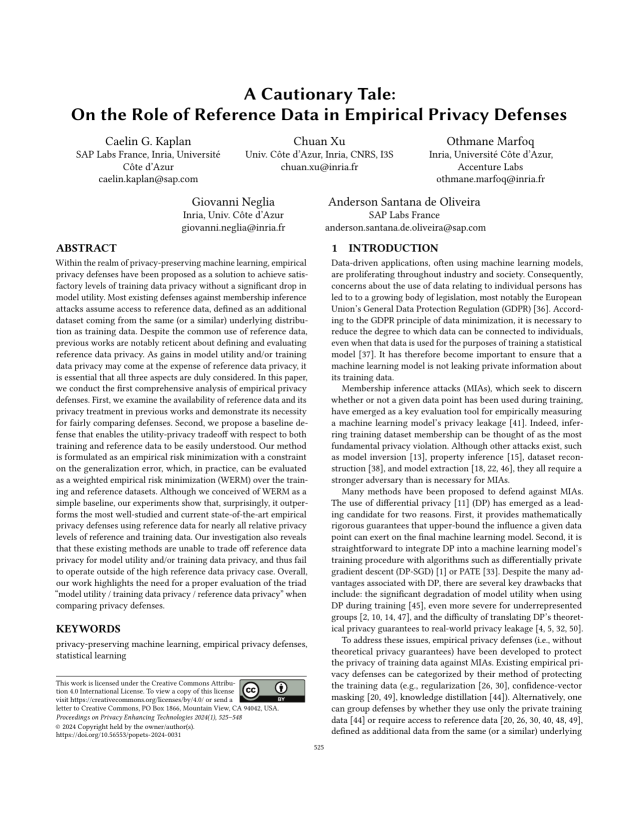