Multiparty Private Set Intersection Cardinality and Its Applications
Authors: Jiahui Gao (Arizona State University), Ni Trieu (Arizona State University), Avishay Yanai (VMware Research)
Volume: 2024
Issue: 2
Pages: 73–90
DOI: https://doi.org/10.56553/popets-2024-0041
Abstract: We describe a new paradigm for multi-party private set intersection cardinality (PSI-CA) that allows $n$ parties to compute the intersection size of their datasets without revealing any additional information. We explore a variety of instantiations of this paradigm. By operating under the assumption that a particular subset of parties refrains from collusion, our protocols avoid computationally expensive public-key operations and are secure in the presence of a semi-honest adversary. We demonstrate the practicality of our PSI-CA with an implementation. For $n=16$ parties with data-sets of $2^{20}$ items each, our server-aided variant takes 71 seconds. Interestingly, in the server-less setting, the same task takes only 7 seconds. To the best of our knowledge, this is the first `special purpose' implementation of a multi-party PSI-CA from symmetric-key techniques (i.e. an implementation that does not rely on a generic underlying MPC).We study two interesting applications -- heatmap computation and associated rule learning (ARL) -- that can be computed securely using a dot-product as a building block. We analyse the performance of securely computing heatmap and ARL using our protocol and compare that to the state-of-the-art.
Keywords: multiparty, private set intersection cardinality, secure dot product
Copyright in PoPETs articles are held by their authors. This article is published under a Creative Commons Attribution 4.0 license.
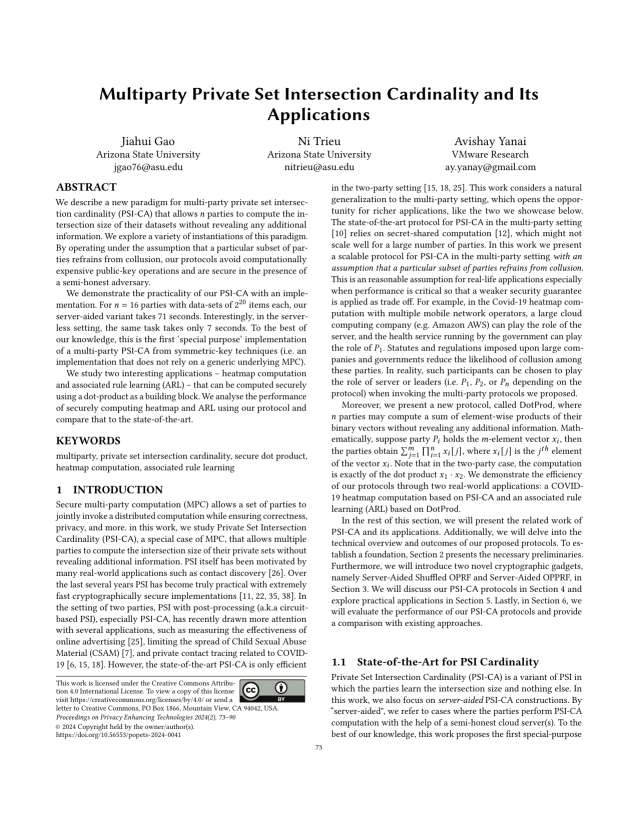