Differentially Private Ad Conversion Measurement
Authors: John Delaney (Google), Badih Ghazi (Google), Charlie Harrison (Google), Christina Ilvento (Google), Ravi Kumar (Google), Pasin Manurangsi (Google), Martin Pál (Google), Karthik Prabhakar (Google), Mariana Raykova (Google)
Volume: 2024
Issue: 2
Pages: 124–140
DOI: https://doi.org/10.56553/popets-2024-0044
Abstract: In this work, we study ad conversion measurement, a central functionality in digital advertising, where an advertiser seeks to estimate advertiser website (or mobile app) conversions attributed to ad impressions that users have interacted with on various publisher websites (or mobile apps). Using differential privacy (DP), a notion that has gained in popularity due to its strong mathematical guarantees, we develop a formal framework for private ad conversion measurement. In particular, we define the notion of an operationally valid configuration of the attribution rule, DP adjacency relation, contribution bounding scope and enforcement point. We then provide, for the set of configurations that most commonly arises in practice, a complete characterization, which uncovers a delicate interplay between attribution and privacy.
Keywords: differential privacy, online advertisement, measurement
Copyright in PoPETs articles are held by their authors. This article is published under a Creative Commons Attribution 4.0 license.
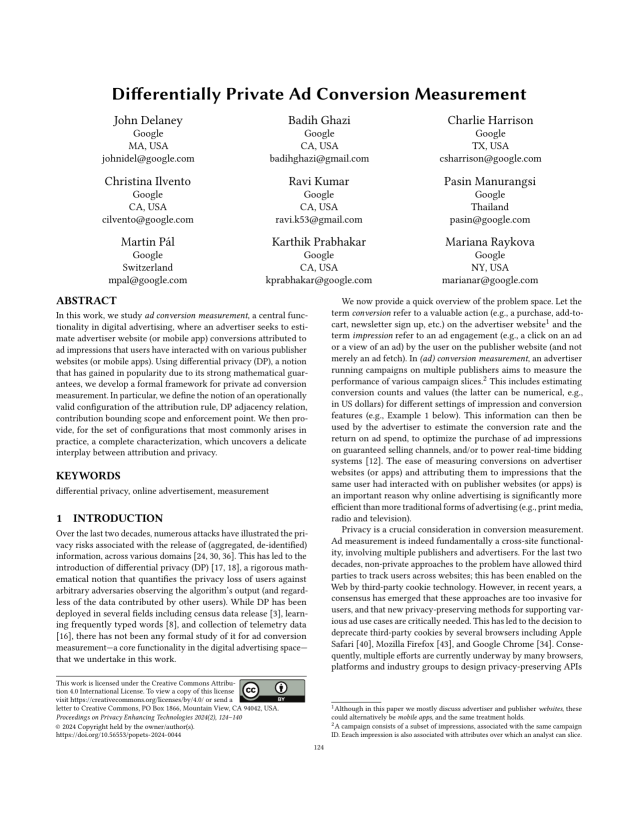