Towards Biologically Plausible and Private Gene Expression Data Generation
Authors: Dingfan Chen (CISPA Helmholtz Center for Information Security), Marie Oestreich (German Center for Neurodegenerative Diseases (DZNE)), Tejumade Afonja (CISPA Helmholtz Center for Information Security), Raouf Kerkouche (CISPA Helmholtz Center for Information Security), Matthias Becker (German Center for Neurodegenerative Diseases (DZNE)), Mario Fritz (CISPA Helmholtz Center for Information Security)
Volume: 2024
Issue: 2
Pages: 531–554
DOI: https://doi.org/10.56553/popets-2024-0062
Abstract: Generative models trained with Differential Privacy (DP) are becoming increasingly prominent in the creation of synthetic data for downstream applications. Existing literature, however, primarily focuses on basic benchmarking datasets and tends to report promising results only for elementary metrics and relatively simple data distributions. In this paper, we initiate a systematic analysis of how DP generative models perform in their natural application scenarios, specifically focusing on real-world gene expression data. We conduct a comprehensive analysis of five representative DP generation methods, examining them from various angles, such as downstream utility, statistical properties, and biological plausibility. Our extensive evaluation illuminates the unique characteristics of each DP generation method, offering critical insights into the strengths and weaknesses of each approach, and uncovering intriguing possibilities for future developments. Perhaps surprisingly, our analysis reveals that most methods are capable of achieving seemingly reasonable downstream utility, according to the standard evaluation metrics considered in existing literature. Nevertheless, we find that none of the DP methods are able to accurately capture the biological characteristics of the real dataset. This observation suggests a potential over-optimistic assessment of current methodologies in this field and underscores a pressing need for future enhancements in model design.
Keywords: gene expression data, differential privacy, data generation, neural networks, synthetic data
Copyright in PoPETs articles are held by their authors. This article is published under a Creative Commons Attribution 4.0 license.
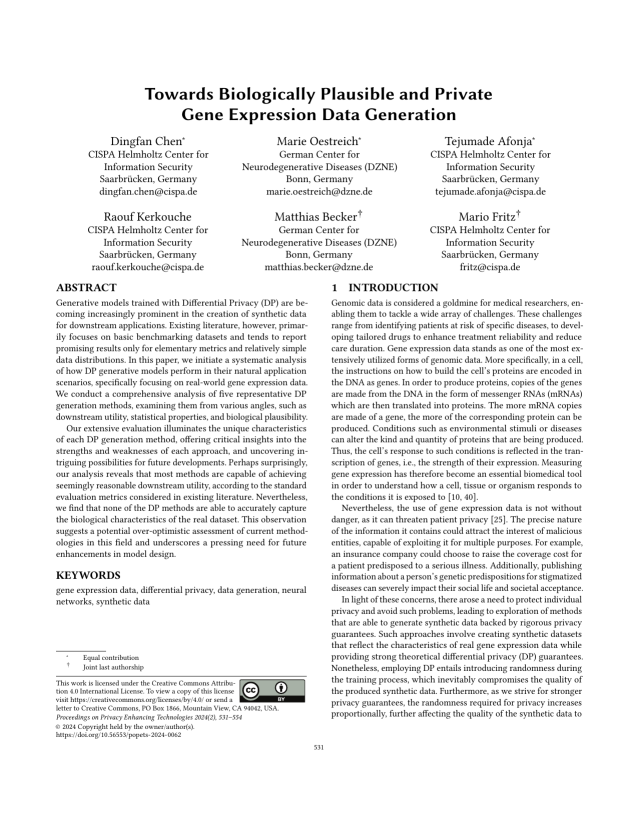