Anonify: Decentralized Dual-level Anonymity for Medical Data Donation
Authors: Sarah Abdelwahab Gaballah (Ruhr University Bochum), Lamya Abdullah (Technical University of Darmstadt), Mina Alishahi (Open Universiteit), Thanh Hoang Long Nguyen (Technical University of Darmstadt), Ephraim Zimmer (Technical University of Darmstadt), Max Mühlhäuser (Technical University of Darmstadt), Karola Marky (Ruhr University Bochum)
Volume: 2024
Issue: 3
Pages: 94–108
DOI: https://doi.org/10.56553/popets-2024-0069
Abstract: Medical data donation involves voluntarily sharing medical data with research institutions, which is crucial for advancing healthcare research. However, the sensitive nature of medical data poses privacy and security challenges. The primary concern is the risk of de-anonymization, where users can be linked to their donated data through background knowledge or communication metadata. In this paper, we introduce Anonify, a decentralized anonymity protocol offering strong user protection during data donation without reliance on a single entity. It achieves dual-level anonymity protection, covering both communication and data aspects by leveraging Distributed Point Functions, and incorporating k-anonymity and stratified sampling within a secret-sharing-based setting. Anonify ensures that the donated data is in a form that affords flexibility for researchers in their analyses. Our evaluation demonstrates the efficiency of Anonify in preserving privacy and optimizing data utility. Furthermore, the performance of machine learning algorithms on the anonymized datasets generated by the protocol shows high accuracy and precision.
Keywords: Medical Data Donation, Data Anonymity, Anonymous Communication, Distributed Point Functions, k-anonymity, Stratified Sampling
Copyright in PoPETs articles are held by their authors. This article is published under a Creative Commons Attribution 4.0 license.
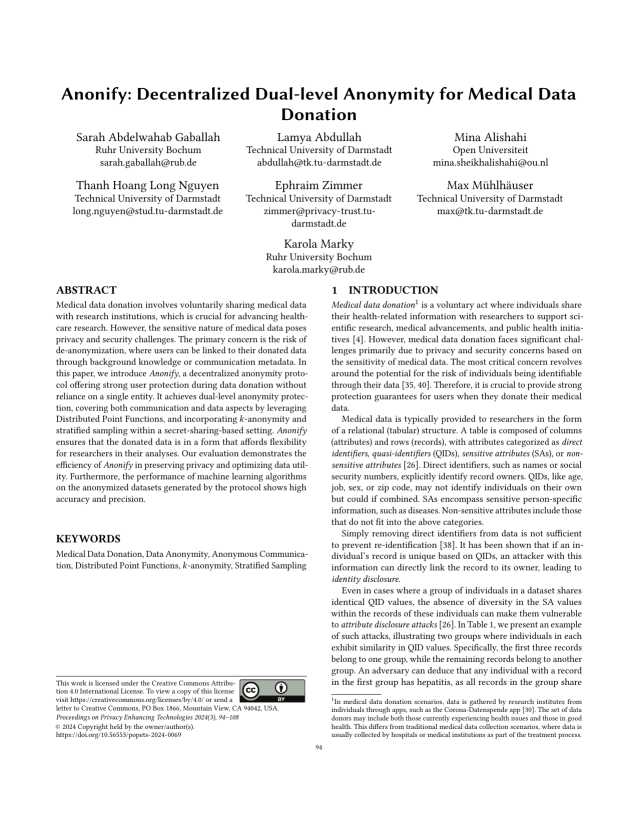