FedLAP-DP: Federated Learning by Sharing Differentially Private Loss Approximations
Authors: Hui-Po Wang (CISPA Helmholtz Center for Information Security), Dingfan Chen (CISPA Helmholtz Center for Information Security), Raouf Kerkouche (CISPA Helmholtz Center for Information Security), Mario Fritz (CISPA Helmholtz Center for Information Security)
Volume: 2024
Issue: 3
Pages: 372–390
DOI: https://doi.org/10.56553/popets-2024-0083
Abstract: Conventional gradient-sharing approaches for federated learning (FL), such as FedAvg, rely on aggregation of local models and often face performance degradation under differential privacy (DP) mechanisms or data heterogeneity, which can be attributed to the inconsistency between the local and global objectives. To address this issue, we propose FedLAP-DP, a novel privacy-preserving approach for FL. Our formulation involves clients synthesizing a small set of samples that approximate local loss landscapes by simulating the gradients of real images within a local region. Acting as loss surrogates, these synthetic samples are aggregated on the server side to uncover the global loss landscape and enable global optimization. Building upon these insights, we offer a new perspective to enforce record-level differential privacy in FL. A formal privacy analysis demonstrates that FedLAP-DP incurs the same privacy costs as typical gradient-sharing schemes while achieving an improved trade-off between privacy and utility. Extensive experiments validate the superiority of our approach across various datasets with highly skewed distributions in both DP and non-DP settings. Beyond the promising performance, our approach presents a faster convergence speed compared to typical gradient-sharing methods and opens up the possibility of trading communication costs for better performance by sending a larger set of synthetic images. The source is available at https://github.com/a514514772/FedLAP-DP.
Keywords: neural networks, federated learning, differential privacy
Copyright in PoPETs articles are held by their authors. This article is published under a Creative Commons Attribution 4.0 license.
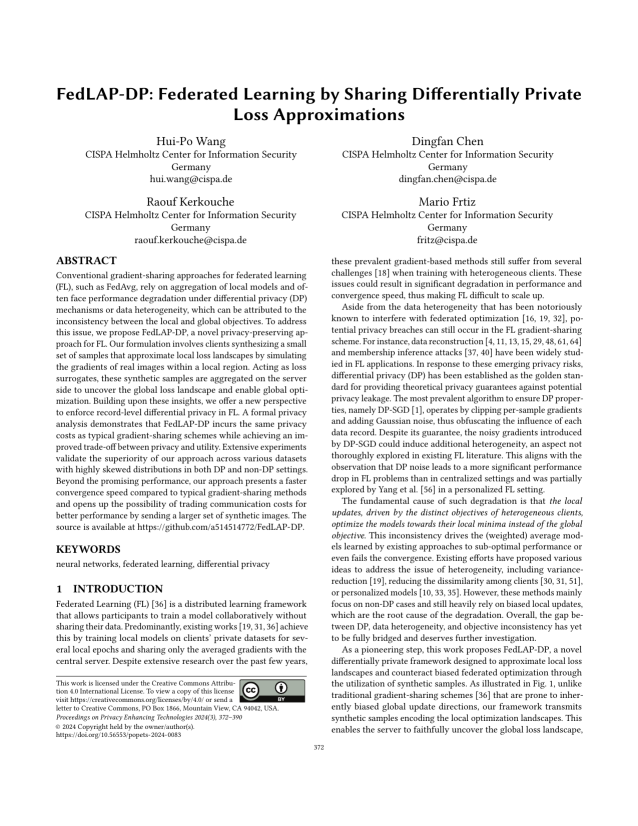