Investigating the Effect of Misalignment on Membership Privacy in the White-box Setting
Authors: Ana-Maria Cretu (EPFL), Daniel Jones (M365 Research), Yves-Alexandre de Montjoye (Imperial College London), Shruti Tople (Azure Research)
Volume: 2024
Issue: 3
Pages: 407–430
DOI: https://doi.org/10.56553/popets-2024-0085
Abstract: Machine learning models have been shown to leak sensitive information about their training datasets. Models are increasingly deployed on devices, raising concerns that white-box access to the model parameters increases the attack surface compared to black-box access which only provides query access. Directly extending the shadow modelling technique from the black-box to the white-box setting has been shown, in general, not to perform better than black-box only attacks. A potential reason is misalignment, a known characteristic of deep neural networks. In the shadow modelling context, misalignment means that, while the shadow models learn similar features in each layer, the features are located in different positions. We here present the first systematic analysis of the causes of misalignment in shadow models and show the use of a different weight initialisation to be the main cause. We then extend several re-alignment techniques, previously developed in the model fusion literature, to the shadow modelling context, where the goal is to re-align the layers of a shadow model to those of the target model.We show re-alignment techniques to significantly reduce the measured misalignment between the target and shadow models. Finally, we perform a comprehensive evaluation of white-box membership inference attacks (MIA). Our analysis reveals that internal layer activation-based MIAs suffer strongly from shadow model misalignment, while gradient-based MIAs are only sometimes significantly affected. We show that re-aligning the shadow models strongly improves the former's performance and can also improve the latter's performance, although less frequently. On the CIFAR10 dataset with a false positive rate of 1%, white-box MIA using re-aligned shadow models improves the true positive rate by 4.5%.Taken together, our results highlight that on-device deployment increases the attack surface and that the newly available information can be used to build more powerful attacks.
Keywords: membership inference attack, machine learning, white-box access, deep neural networks, symmetries, privacy
Copyright in PoPETs articles are held by their authors. This article is published under a Creative Commons Attribution 4.0 license.
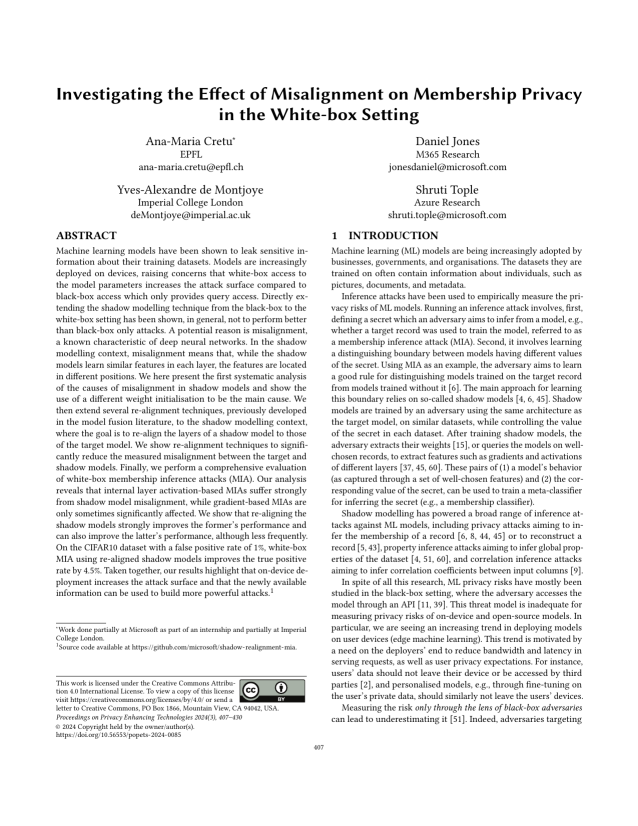