A Zero Auxiliary Knowledge Membership Inference Attack on Aggregate Location Data
Authors: Vincent Guan (Imperial College London), Florent Guépin (Imperial College London), Ana-Maria Cretu (EPFL), Yves-Alexandre de Montjoye (Imperial College London)
Volume: 2024
Issue: 4
Pages: 80–101
DOI: https://doi.org/10.56553/popets-2024-0108
Abstract: Location data is frequently collected from populations and shared in aggregate form to guide policy and decision making. However, the prevalence of aggregated data also raises the privacy concern of membership inference attacks (MIAs). MIAs infer whether an individual's data contributed to the aggregate release. Although effective MIAs have been developed for aggregate location data, these require access to an extensive auxiliary dataset of individual traces over the same locations, which are collected from a similar population. This assumption is often impractical given common privacy practices surrounding location data. To measure the risk of an MIA performed by a realistic adversary, we develop the first Zero Auxiliary Knowledge (ZK) MIA on aggregate location data, which eliminates the need for an auxiliary dataset of real individual traces. Instead, we develop a novel synthetic approach, such that suitable synthetic traces are generated from the released aggregate. We also develop methods to correct for bias and noise, to show that our synthetic-based attack is still applicable when privacy mechanisms are applied prior to release. Using two large-scale location datasets, we demonstrate that our ZK MIA matches the state-of-the-art Knock-Knock (KK) MIA across a wide range of settings, including popular implementations of differential privacy (DP) and suppression of small counts. Furthermore, we show that ZK MIA remains highly effective even when the adversary only knows a small fraction (10%) of their target's location history. This demonstrates that effective MIAs can be performed by realistic adversaries, highlighting the need for strong DP protection.
Keywords: Location data, Membership inference attack, Synthetic data
Copyright in PoPETs articles are held by their authors. This article is published under a Creative Commons Attribution 4.0 license.
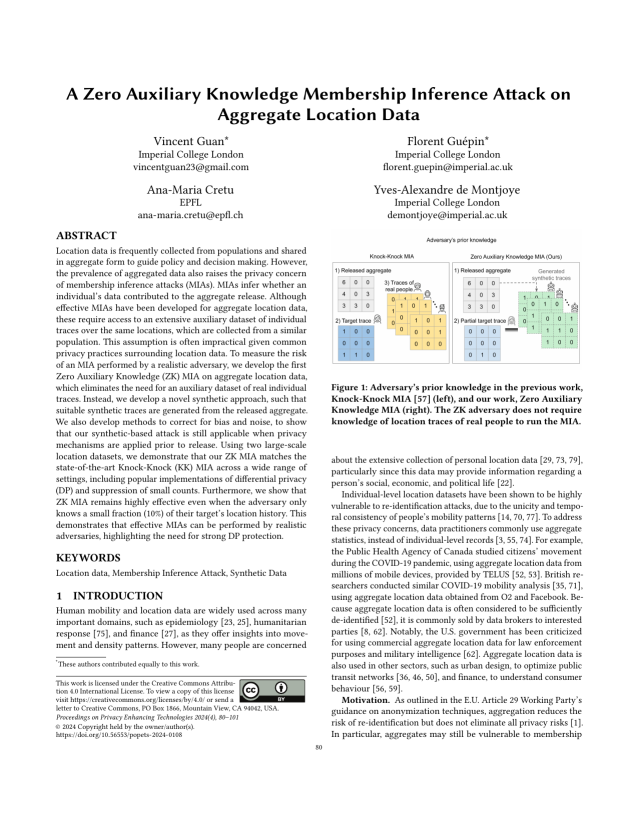