Diversity-driven Privacy Protection Masks Against Unauthorized Face Recognition
Authors: Ka-Ho Chow (The University of Hong Kong), Sihao Hu (Georgia Institute of Technology), Tiansheng Huang (Georgia Institute of Technology), Fatih Ilhan (Georgia Institute of Technology), Wenqi Wei (Georgia Institute of Technology), Ling Liu (Georgia Institute of Technology)
Volume: 2024
Issue: 4
Pages: 381–392
DOI: https://doi.org/10.56553/popets-2024-0122
Abstract: Face recognition (FR) technologies have enabled many life-enriching applications but have also opened doors for potential misuse. Governments, private companies, or even individuals can scrape the web, collect facial images, and build a face database to fuel the FR system to identify human faces without their consent. This paper introduces PMask to combat such a privacy threat against unauthorized FR. It provides a holistic approach to enable privacy-preserving sharing of facial images. PMask preprocesses the facial image and hides its unique facial signature through iterative optimization with dual goals: (i) minimizing the amount of noise to ensure high image quality and (ii) minimizing the perception loss between the privacy-protected face and the original face to ensure the face is recognizable to be the same person by humans. Extensive experiments are conducted on eight representative FR models to evaluate PMask against unauthorized FR. The results validate that PMask provides much stronger protection, introduces less perceptible changes to facial images, and runs faster than state-of-the-art methods to provide privacy protection with a better user experience.
Keywords: privacy, face recognition, neural networks
Copyright in PoPETs articles are held by their authors. This article is published under a Creative Commons Attribution 4.0 license.
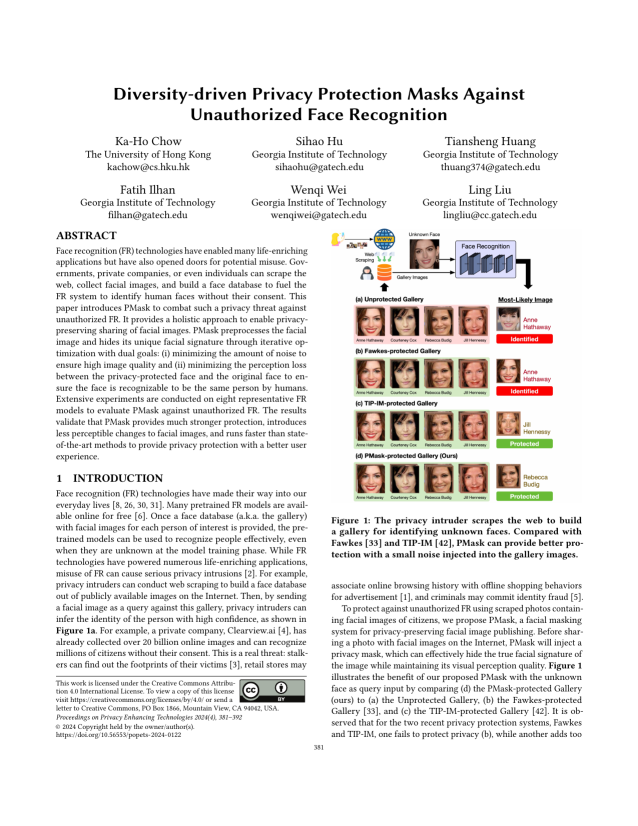