Crowdsourcing the Discovery of Server-side Censorship Evasion Strategies
Authors: Nhi Tran (University of Maryland), Kevin Bock (University of Maryland), Dave Levin (University of Maryland)
Year: 2023
Issue: 2
Pages: 88–90
Abstract: Nation-state censorship affects billions of people globally, limiting their access to new information and viewpoints. While regimes enhance their censorship infrastructure, researchers continue to develop tools and techniques to evade such restrictions. One such recent method is server-side censorship evasion, in which the server modifies the packet stream during a TCP three-way handshake to bypass censorship on behalf of the client. Automated systems to discover server-side evasion strategies (such as Geneva) are limited in that they require instrumented clients inside of censored regimes to issue requests to the server. In this extended abstract, we propose a design for how we can discover censorship evasion strategies without directly instrumenting client participation in training. We suggest a distributed training model where users can visit a website using an unmodified web browser and contribute to censorship evasion training (after providing informed consent). We discuss the challenges and limitations of constructing such a distributed training system.
Copyright in FOCI articles are held by their authors. This article is published under a Creative Commons Attribution 4.0 license.
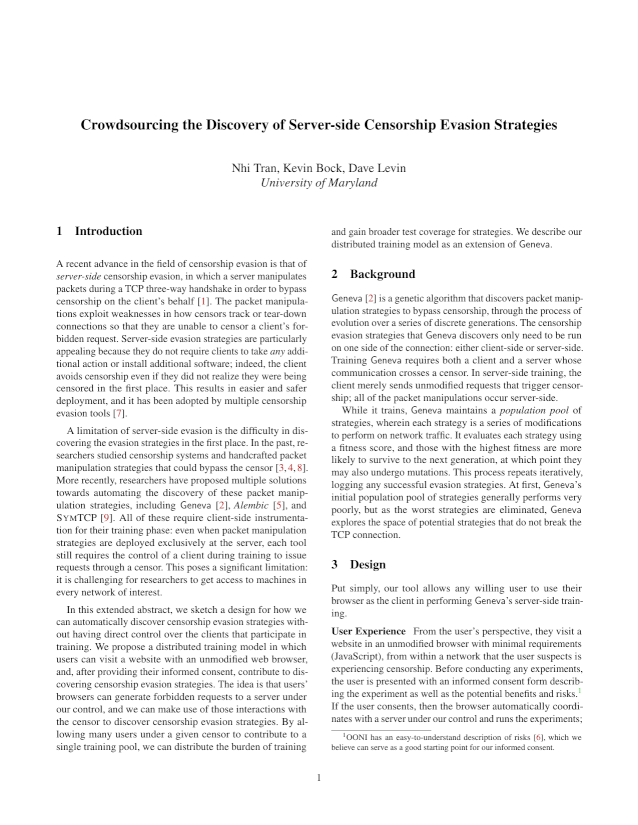