Extended Abstract: The Impact of Online Censorship on LLMs
Authors: Mohamed Ahmed (Citizen Lab, University of Toronto), Jeffrey Knockel (Citizen Lab, University of Toronto)
Year: 2024
Issue: 2
Pages: 1–9
Abstract: While there has been a substantial and growing effort to identify, analyze, and mitigate implicit biases in large language models (LLMs), little emphasis has been put on measuring the impacts of online censorship practices on these models. In the same way that biases and false information ingrained in training datasets may manifest themselves implicitly in the outputs of models, training a generative model on censored content, i.e., content that is subject to censorship rules, may impact the views reflected in its responses. Furthermore, the issue may be accentuated for speakers of a language if a substantial portion of a model’s training data in that language has been subject to censorship. We propose an experiment for analyzing the effects of online censorship on black-box LLMs by evaluating models' responses to prompts made in Simplified and Traditional Chinese to determine their similarity to known censored content. Our exploratory testing suggests that, when asked in Simplified Chinese, LLMs provide answers largely in keeping with Chinese information control requirements unlike when asked in Traditional Chinese. Due to the popular usage of LLMs globally, we hypothesize that LLMs unwittingly export information manipulation that would have primarily harmed a domestic audience to diaspora and other Chinese speakers living abroad.
Copyright in FOCI articles are held by their authors. This article is published under a Creative Commons Attribution 4.0 license.
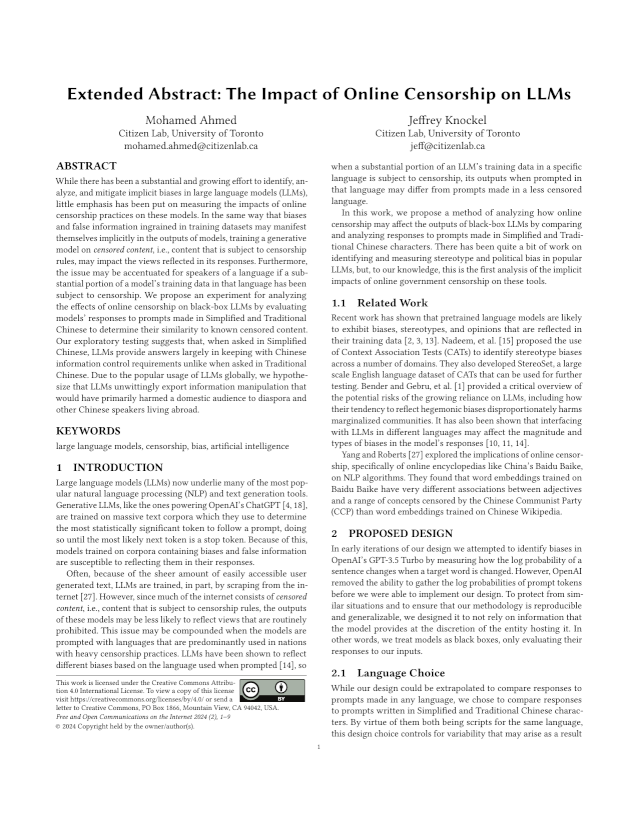