Extended Abstract: Leveraging Large Language Models to Identify Internet Censorship through Network Data
Authors: Tianyu Gao (Graduate Center, City University of New York), Ping Ji (Graduate Center, City University of New York)
Year: 2024
Issue: 2
Pages: 10–12
Abstract: With the intensification of internet censorship measures implemented across the globe, detecting and analyzing censorship events have become crucial for preserving Internet freedom. The network reachability data collected by existing censorship monitoring platforms such as OONI, Censored Planet, and ICLab offers a comprehensive and longitudinal view of global censorship efforts but presents challenges in data analysis with growing data volume and complexity. In this study, we aim to navigate a novel approach to detect internet censorship by applying Large Language Models (LLMs) to analyze network reachability data, with the goal of mitigating challenges posed by the massive volumes of data collected by the platforms. We explore the potential of LLMs, such as GPT-3 and BERT, on processing and interpreting the extensive, complex network data to identify patterns indicative of censorship. By integrating innovative LLM methodologies with data collected from existing censorship monitoring platforms, we hope to enhance the accuracy and scalability of censorship detection, so that more robust defense strategies can be developed
Copyright in FOCI articles are held by their authors. This article is published under a Creative Commons Attribution 4.0 license.
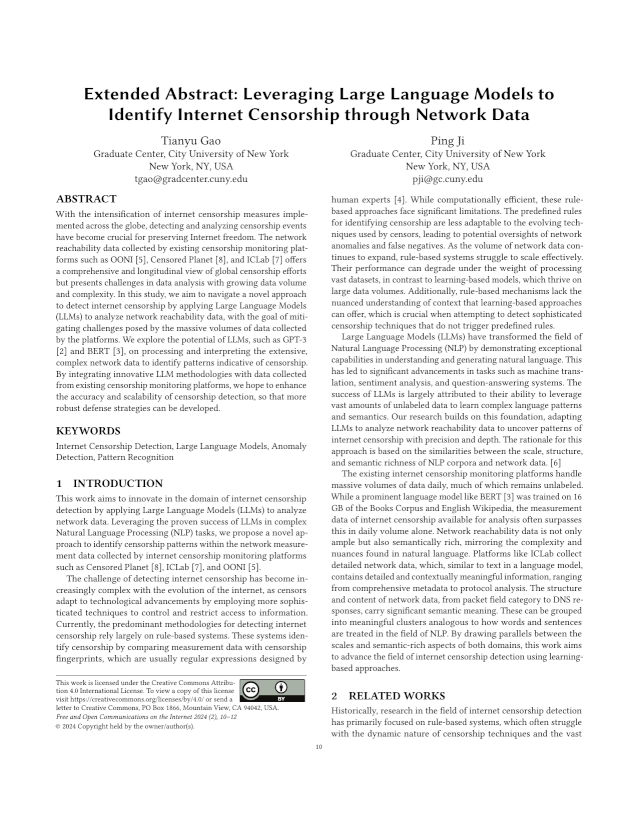