Near-Optimal Fingerprinting with Constraints
Authors: Gábor György Gulyás (INRIA), Gergely Acs (INRIA), Claude Castelluccia (INRIA)
Volume: 2016
Issue: 4
Pages: 470–487
DOI: https://doi.org/10.1515/popets-2016-0051
Abstract: Several recent studies have demonstrated that people show large behavioural uniqueness. This has serious privacy implications as most individuals become increasingly re-identifiable in large datasets or can be tracked, while they are browsing the web, using only a couple of their attributes, called as their fingerprints. Often, the success of these attacks depends on explicit constraints on the number of attributes learnable about individuals, i.e., the size of their fingerprints. These constraints can be budget as well as technical constraints imposed by the data holder. For instance, Apple restricts the number of applications that can be called by another application on iOS in order to mitigate the potential privacy threats of leaking the list of installed applications on a device. In this work, we address the problem of identifying the attributes (e.g., smartphone applications) that can serve as a fingerprint of users given constraints on the size of the fingerprint. We give the best fingerprinting algorithms in general, and evaluate their effectiveness on several real-world datasets. Our results show that current privacy guards limiting the number of attributes that can be queried about individuals is insufficient to mitigate their potential privacy risks in many practical cases.
Copyright in PoPETs articles are held by their authors. This article is published under a Creative Commons Attribution-NonCommercial-NoDerivs 3.0 license.
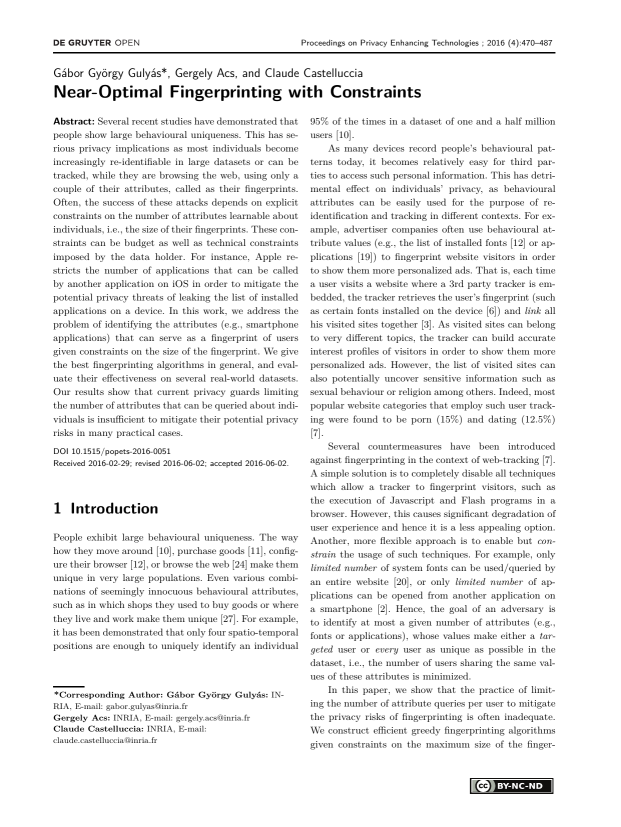