Olympus: Sensor Privacy through Utility Aware Obfuscation
Authors: Nisarg Raval (Duke University), Ashwin Machanavajjhala (Duke University), Jerry Pan (Duke University)
Volume: 2019
Issue: 1
Pages: 5–25
DOI: https://doi.org/10.2478/popets-2019-0002
Abstract: Personal data garnered from various sensors are often offloaded by applications to the cloud for analytics. This leads to a potential risk of disclosing private user information. We observe that the analytics run on the cloud are often limited to a machine learning model such as predicting a user’s activity using an activity classifier. We present Olympus, a privacy framework that limits the risk of disclosing private user information by obfuscating sensor data while minimally affecting the functionality the data are intended for. Olympus achieves privacy by designing a utility aware obfuscation mechanism, where privacy and utility requirements are modeled as adversarial networks. By rigorous and comprehensive evaluation on a real world app and on benchmark datasets, we show that Olympus successfully limits the disclosure of private information without significantly affecting functionality of the application.
Keywords: machine learning, mobile, privacy
Copyright in PoPETs articles are held by their authors. This article is published under a Creative Commons Attribution-NonCommercial-NoDerivs 3.0 license.
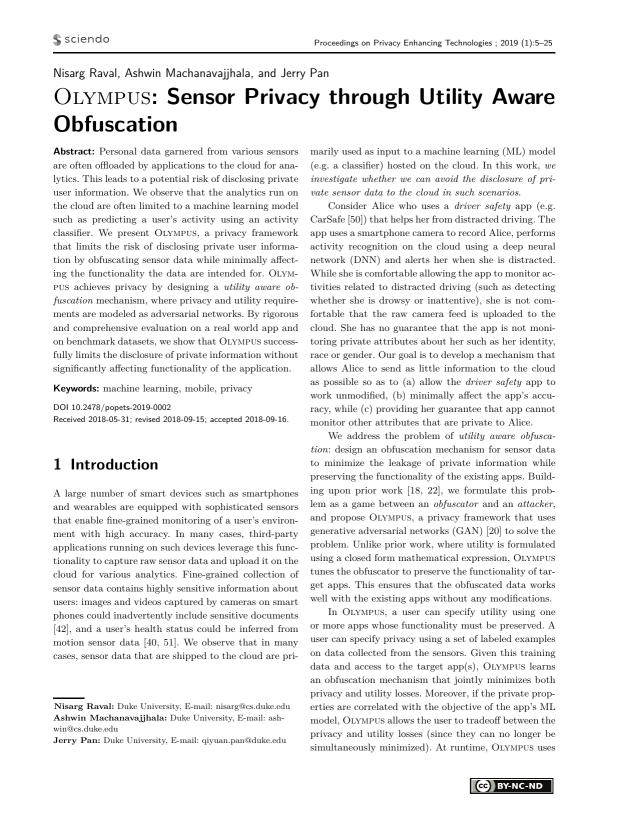