RON-Gauss: Enhancing Utility in Non-Interactive Private Data Release
Authors: Thee Chanyaswad (Princeton University), Changchang Liu (Princeton University), Prateek Mittal (Princeton University)
Volume: 2019
Issue: 1
Pages: 26–46
DOI: https://doi.org/10.2478/popets-2019-0003
Abstract: A key challenge facing the design of differential privacy in the non-interactive setting is to maintain the utility of the released data. To overcome this challenge, we utilize the Diaconis-Freedman-Meckes (DFM) effect, which states that most projections of high-dimensional data are nearly Gaussian. Hence, we propose the RON-Gauss model that leverages the novel combination of dimensionality reduction via random orthonormal (RON) projection and the Gaussian generative model for synthesizing differentially-private data. We analyze how RON-Gauss benefits from the DFM effect, and present multiple algorithms for a range of machine learning applications, including both unsupervised and supervised learning. Furthermore, we rigorously prove that (a) our algorithms satisfy the strong -differential privacy guarantee, and (b) RON projection can lower the level of perturbation required for differential privacy. Finally, we illustrate the effectiveness of RON-Gauss under three common machine learning applications – clustering, classification, and regression – on three large real-world datasets. Our empirical results show that (a) RON-Gauss outperforms previous approaches by up to an order of magnitude, and (b) loss in utility compared to the non-private real data is small. Thus, RON-Gauss can serve as a key enabler for realworld deployment of privacy-preserving data release.
Keywords: differential privacy, non-interactive private data release, random orthonormal projection, Gaussian generative model, Diaconis-Freedman-Meckes effect
Copyright in PoPETs articles are held by their authors. This article is published under a Creative Commons Attribution-NonCommercial-NoDerivs 3.0 license.
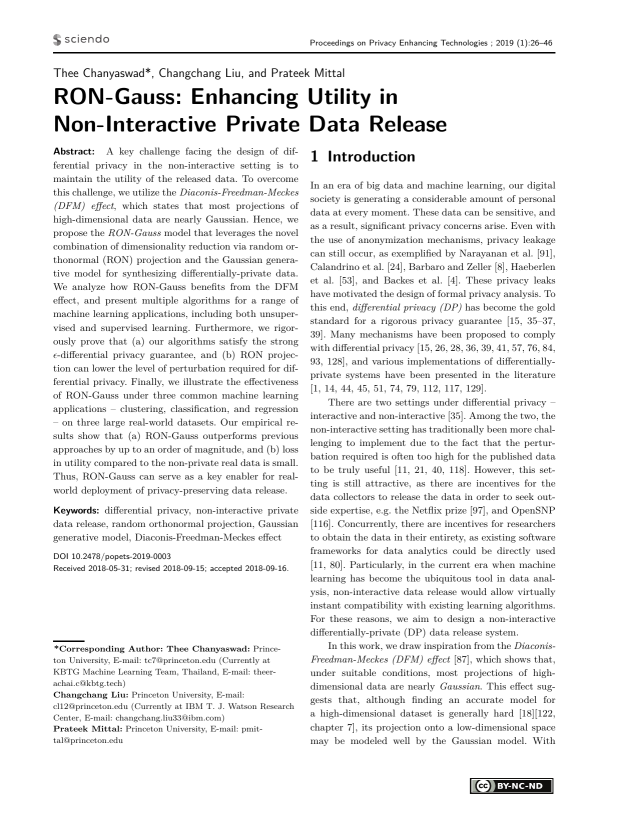