SoK: Privacy-Preserving Collaborative Tree-based Model Learning
Authors: Sylvain Chatel (Laboratory for Data Security – EPFL), Apostolos Pyrgelis (Laboratory for Data Security – EPFL), Juan Ramón Troncoso-Pastoriza (Laboratory for Data Security – EPFL), Jean-Pierre Hubaux (Laboratory for Data Security – EPFL)
Volume: 2021
Issue: 3
Pages: 182–203
DOI: https://doi.org/10.2478/popets-2021-0043
Abstract: Tree-based models are among the most efficient machine learning techniques for data mining nowadays due to their accuracy, interpretability, and simplicity. The recent orthogonal needs for more data and privacy protection call for collaborative privacy-preserving solutions. In this work, we survey the literature on distributed and privacy-preserving training of tree-based models and we systematize its knowledge based on four axes: the learning algorithm, the collaborative model, the protection mechanism, and the threat model. We use this to identify the strengths and limitations of these works and provide for the first time a framework analyzing the information leakage occurring in distributed tree-based model learning.
Keywords: decision-tree induction, collaborative learning, privacy-preserving protocols, leakage analysis
Copyright in PoPETs articles are held by their authors. This article is published under a Creative Commons Attribution-NonCommercial-NoDerivs 3.0 license.
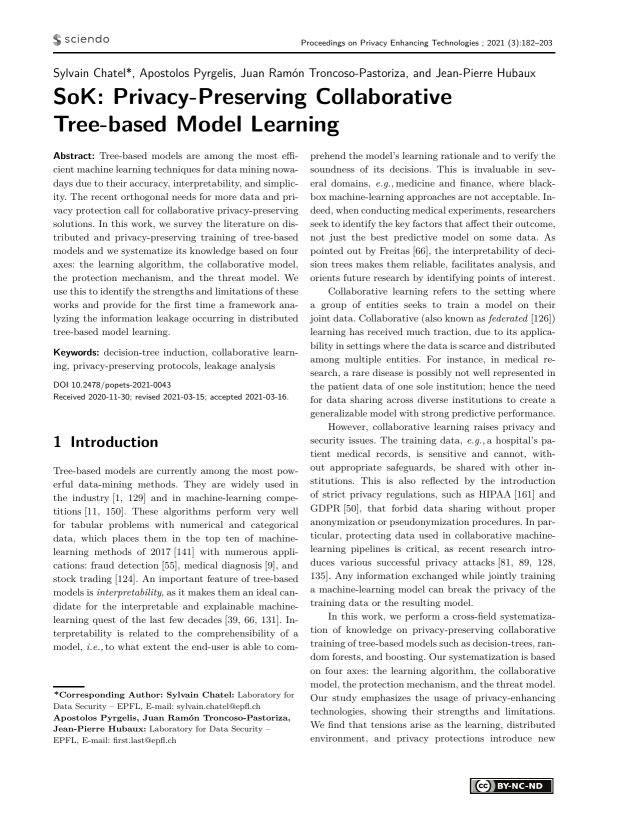