FoggySight: A Scheme for Facial Lookup Privacy
Authors: Ivan Evtimov (Paul G. Allen School of Computer Science & Engineering, University of Washington), Pascal Sturmfels (Paul G. Allen School of Computer Science & Engineering, University of Washington), Tadayoshi Kohno (Paul G. Allen School of Computer Science & Engineering, University of Washington)
Volume: 2021
Issue: 3
Pages: 204–226
DOI: https://doi.org/10.2478/popets-2021-0044
Abstract: Advances in deep learning algorithms have enabled better-than-human performance on face recognition tasks. In parallel, private companies have been scraping social media and other public websites that tie photos to identities and have built up large databases of labeled face images. Searches in these databases are now being offered as a service to law enforcement and others and carry a multitude of privacy risks for social media users. In this work, we tackle the problem of providing privacy from such face recognition systems. We propose and evaluate FoggySight, a solution that applies lessons learned from the adversarial examples literature to modify facial photos in a privacy-preserving manner before they are uploaded to social media. FoggySight’s core feature is a community protection strategy where users acting as protectors of privacy for others upload decoy photos generated by adversarial machine learning algorithms. We explore different settings for this scheme and find that it does enable protection of facial privacy – including against a facial recognition service with unknown internals.
Keywords: facial recognition, privacy, adversarial examples, deep learning
Copyright in PoPETs articles are held by their authors. This article is published under a Creative Commons Attribution-NonCommercial-NoDerivs 3.0 license.
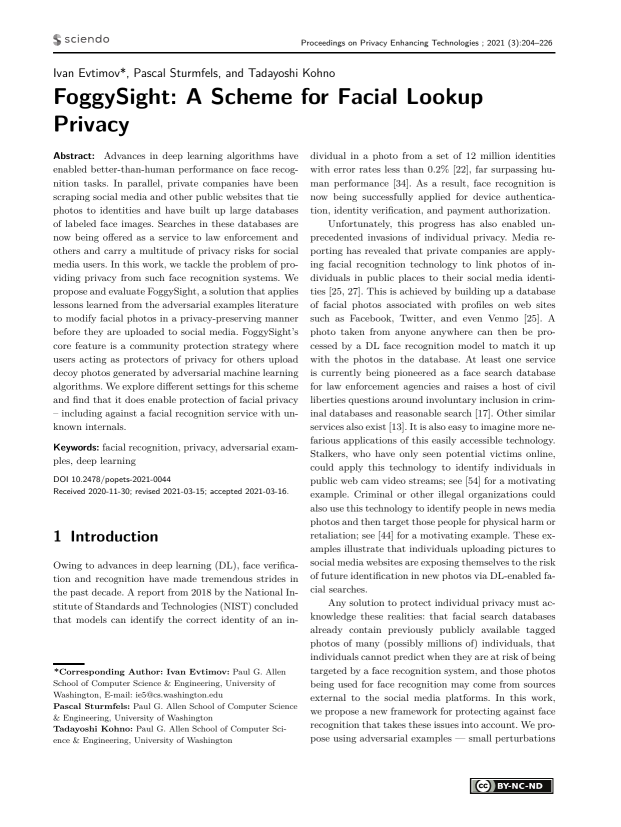