Disparate Vulnerability to Membership Inference Attacks
Authors: Bogdan Kulynych (EPFL), Mohammad Yaghini (University of Toronto, Vector Institute), Giovanni Cherubin (Alan Turing Institute), Michael Veale (University College London), Carmela Troncoso (EPFL)
Volume: 2022
Issue: 1
Pages: 460–480
DOI: https://doi.org/10.2478/popets-2022-0023
Abstract: A membership inference attack (MIA) against a machine-learning model enables an attacker to determine whether a given data record was part of the model’s training data or not. In this paper, we provide an in-depth study of the phenomenon of disparate vulnerability against MIAs: unequal success rate of MIAs against different population subgroups. We first establish necessary and sufficient conditions for MIAs to be prevented, both on average and for population subgroups, using a notion of distributional generalization. Second, we derive connections of disparate vulnerability to algorithmic fairness and to differential privacy. We show that fairness can only prevent disparate vulnerability against limited classes of adversaries. Differential privacy bounds disparate vulnerability but can significantly reduce the accuracy of the model. We show that estimating disparate vulnerability by naïvely applying existing attacks can lead to overestimation. We then establish which attacks are suitable for estimating disparate vulnerability, and provide a statistical framework for doing so reliably. We conduct experiments on synthetic and real-world data finding significant evidence of disparate vulnerability in realistic settings.
Keywords: membership inference attacks, machine learning, fairness
Copyright in PoPETs articles are held by their authors. This article is published under a Creative Commons Attribution-NonCommercial-NoDerivs 3.0 license.
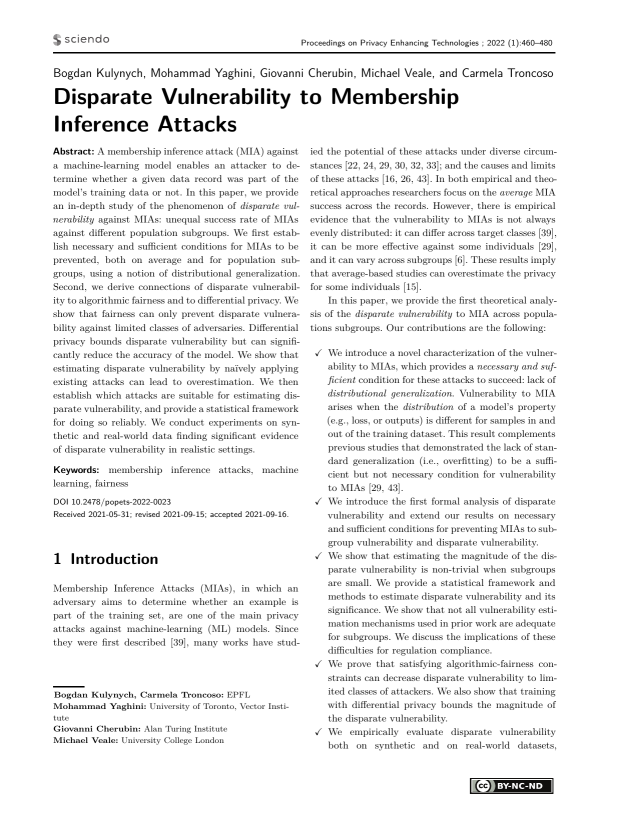