Privacy-Preserving High-dimensional Data Collection with Federated Generative Autoencoder
Authors: Xue Jiang (Technische Universität München, Huawei Technologies Düsseldorf GmbH), Xuebing Zhou (Huawei Technologies Düsseldorf GmbH), Jens Grossklags (Technische Universität München)
Volume: 2022
Issue: 1
Pages: 481–500
DOI: https://doi.org/10.2478/popets-2022-0024
Abstract: Business intelligence and AI services often involve the collection of copious amounts of multidimensional personal data. Since these data usually contain sensitive information of individuals, the direct collection can lead to privacy violations. Local differential privacy (LDP) is currently considered a state-ofthe-art solution for privacy-preserving data collection. However, existing LDP algorithms are not applicable to high-dimensional data; not only because of the increase in computation and communication cost, but also poor data utility. In this paper, we aim at addressing the curse-ofdimensionality problem in LDP-based high-dimensional data collection. Based on the idea of machine learning and data synthesis, we propose DP-Fed-Wae, an efficient privacy-preserving framework for collecting highdimensional categorical data. With the combination of a generative autoencoder, federated learning, and differential privacy, our framework is capable of privately learning the statistical distributions of local data and generating high utility synthetic data on the server side without revealing users’ private information. We have evaluated the framework in terms of data utility and privacy protection on a number of real-world datasets containing 68–124 classification attributes. We show that our framework outperforms the LDP-based baseline algorithms in capturing joint distributions and correlations of attributes and generating high-utility synthetic data. With a local privacy guarantee = 8, the machine learning models trained with the synthetic data generated by the baseline algorithm cause an accuracy loss of 10% ∼ 30%, whereas the accuracy loss is significantly reduced to less than 3% and at best even less than 1% with our framework. Extensive experimental results demonstrate the capability and efficiency of our framework in synthesizing high-dimensional data while striking a satisfactory utility-privacy balance.
Keywords: high-dimensional data collection, local differential privacy, federated learning, generative models
Copyright in PoPETs articles are held by their authors. This article is published under a Creative Commons Attribution-NonCommercial-NoDerivs 3.0 license.
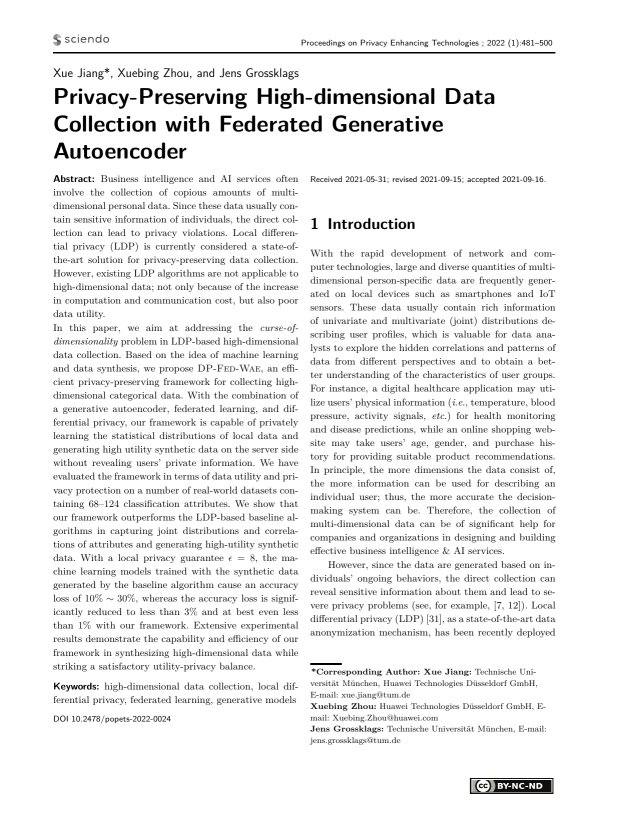