Understanding Utility and Privacy of Demographic Data in Education Technology by Causal Analysis and Adversarial-Censoring
Authors: Rakibul Hasan (Arizona State University), Mario Fritz (CISPA Helmholtz Center for Information Security)
Volume: 2022
Issue: 2
Pages: 245–262
DOI: https://doi.org/10.2478/popets-2022-0044
Abstract: Education technologies (EdTech) are becoming pervasive due to their cost-effectiveness, accessibility, and scalability. They also experienced accelerated market growth during the recent pandemic. EdTech collects massive amounts of students’ behavioral and (sensitive) demographic data, often justified by the potential to help students by personalizing education. Researchers voiced concerns regarding privacy and data abuses (e.g., targeted advertising) in the absence of clearly defined data collection and sharing policies. However, technical contributions to alleviating students’ privacy risks have been scarce. In this paper, we argue against collecting demographic data by showing that gender—a widely used demographic feature—does not causally affect students’ course performance: arguably the most popular target of predictive models. Then, we show that gender can be inferred from behavioral data; thus, simply leaving them out does not protect students’ privacy. Combining a feature selection mechanism with an adversarial censoring technique, we propose a novel approach to create a ‘private’ version of a dataset comprising of fewer features that predict the target without revealing the gender, and are interpretive. We conduct comprehensive experiments on a public dataset to demonstrate the robustness and generalizability of our mechanism.
Keywords: Privacy, Educational data mining, Learning analytics, Educational Technology
Copyright in PoPETs articles are held by their authors. This article is published under a Creative Commons Attribution-NonCommercial-NoDerivs 3.0 license.
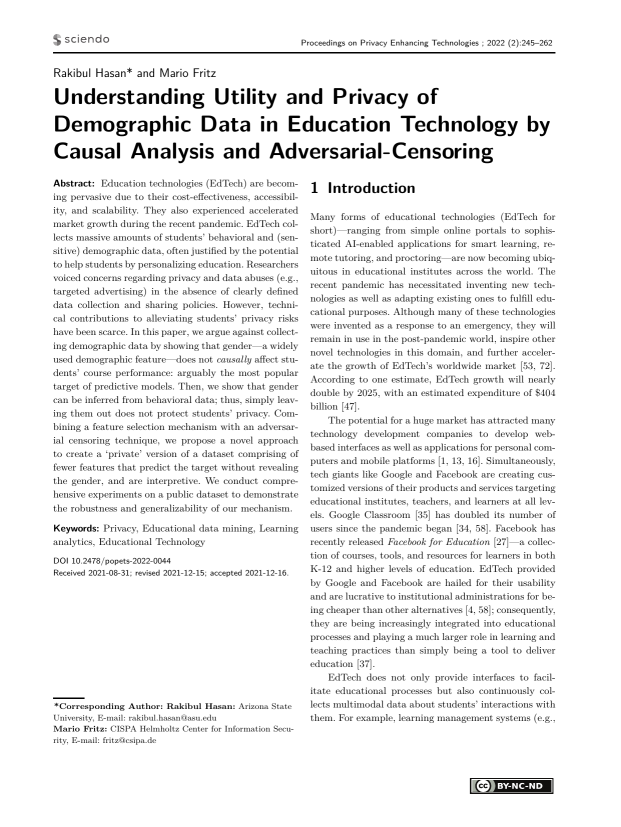